Open Access
ARTICLE
Crop Disease Recognition Based on Improved Model-Agnostic Meta-Learning
1 College of Information Technology, Jilin Agricultural University, Changchun, 130118, China
2 College of Engineering and Technology, Jilin Agricultural University, Changchun, 130118, China
* Corresponding Author: Lidong Chu. Email:
Computers, Materials & Continua 2023, 75(3), 6101-6118. https://doi.org/10.32604/cmc.2023.036829
Received 13 October 2022; Accepted 08 February 2023; Issue published 29 April 2023
Abstract
Currently, one of the most severe problems in the agricultural industry is the effect of diseases and pests on global crop production and economic development. Therefore, further research in the field of crop disease and pest detection is necessary to address the mentioned problem. Aiming to identify the diseased crops and insect pests timely and accurately and perform appropriate prevention measures to reduce the associated losses, this article proposes a Model-Agnostic Meta-Learning (MAML) attention model based on the meta-learning paradigm. The proposed model combines meta-learning with basic learning and adopts an Efficient Channel Attention (ECA) module. The module follows the local cross-channel interactive strategy of non-dimensional reduction to strengthen the weight parameters corresponding to certain disease characteristics. The proposed meta-learning-based algorithm has the advantage of strong generalization capability and, by integrating the ECA module in the original model, can achieve more efficient detection in new tasks. The proposed model is verified by experiments, and the experimental results show that compared with the original MAML model, the proposed improved MAML-Attention model has a better performance by 1.8–9.31 percentage points in different classification tasks; the maximum accuracy is increased by 1.15–8.2 percentage points. The experimental results verify the strong generalization ability and good robustness of the proposed MAML-Attention model. Compared to the other few-shot methods, the proposed MAML-Attention performs better.Keywords
Cite This Article
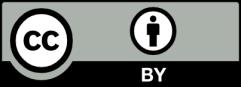
This work is licensed under a Creative Commons Attribution 4.0 International License , which permits unrestricted use, distribution, and reproduction in any medium, provided the original work is properly cited.