Open Access
ARTICLE
Ensemble Deep Learning Framework for Situational Aspects-Based Annotation and Classification of International Student’s Tweets during COVID-19
1 School of Information Engineering, Zhengzhou University, Zhengzhou, China
2 School of Computer Science and Engineering, Central South University, Changsha, China
3 Department of Data Science, The Islamia University of Bahawalpur, Bahawalpur, Pakistan
4 Clausthal University of Technology, Clausthal-Zellerfeld, Germany
* Corresponding Author: Hou Weiyan. Email:
Computers, Materials & Continua 2023, 75(3), 5355-5377. https://doi.org/10.32604/cmc.2023.036779
Received 12 October 2022; Accepted 29 January 2023; Issue published 29 April 2023
Abstract
As the COVID-19 pandemic swept the globe, social media platforms became an essential source of information and communication for many. International students, particularly, turned to Twitter to express their struggles and hardships during this difficult time. To better understand the sentiments and experiences of these international students, we developed the Situational Aspect-Based Annotation and Classification (SABAC) text mining framework. This framework uses a three-layer approach, combining baseline Deep Learning (DL) models with Machine Learning (ML) models as meta-classifiers to accurately predict the sentiments and aspects expressed in tweets from our collected Student-COVID-19 dataset. Using the proposed aspect2class annotation algorithm, we labeled bulk unlabeled tweets according to their contained aspect terms. However, we also recognized the challenges of reducing data’s high dimensionality and sparsity to improve performance and annotation on unlabeled datasets. To address this issue, we proposed the Volatile Stopwords Filtering (VSF) technique to reduce sparsity and enhance classifier performance. The resulting Student-COVID Twitter dataset achieved a sophisticated accuracy of 93.21% when using the random forest as a meta-classifier. Through testing on three benchmark datasets, we found that the SABAC ensemble framework performed exceptionally well. Our findings showed that international students during the pandemic faced various issues, including stress, uncertainty, health concerns, financial stress, and difficulties with online classes and returning to school. By analyzing and summarizing these annotated tweets, decision-makers can better understand and address the real-time problems international students face during the ongoing pandemic.Keywords
Cite This Article
S. Hussain, M. Ayoub, Y. Yu, J. A. Wahid, A. Khan et al., "Ensemble deep learning framework for situational aspects-based annotation and classification of international student’s tweets during covid-19," Computers, Materials & Continua, vol. 75, no.3, pp. 5355–5377, 2023. https://doi.org/10.32604/cmc.2023.036779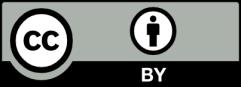