Open Access
ARTICLE
APST-Flow: A Reversible Network-Based Artistic Painting Style Transfer Method
Faculty of Information Engineering and Automation, Kunming University of Science and Technology, Kunming, 650500, China
* Corresponding Author: Meng Wang. Email:
Computers, Materials & Continua 2023, 75(3), 5229-5254. https://doi.org/10.32604/cmc.2023.036631
Received 07 October 2022; Accepted 03 March 2023; Issue published 29 April 2023
Abstract
In recent years, deep generative models have been successfully applied to perform artistic painting style transfer (APST). The difficulties might lie in the loss of reconstructing spatial details and the inefficiency of model convergence caused by the irreversible en-decoder methodology of the existing models. Aiming to this, this paper proposes a Flow-based architecture with both the en-decoder sharing a reversible network configuration. The proposed APST-Flow can efficiently reduce model uncertainty via a compact analysis-synthesis methodology, thereby the generalization performance and the convergence stability are improved. For the generator, a Flow-based network using Wavelet additive coupling (WAC) layers is implemented to extract multi-scale content features. Also, a style checker is used to enhance the global style consistency by minimizing the error between the reconstructed and the input images. To enhance the generated salient details, a loss of adaptive stroke edge is applied in both the global and local model training. The experimental results show that the proposed method improves PSNR by 5%, SSIM by 6.2%, and decreases Style Error by 29.4% over the existing models on the ChipPhi set. The competitive results verify that APST-Flow achieves high-quality generation with less content deviation and enhanced generalization, thereby can be further applied to more APST scenes.Keywords
Cite This Article
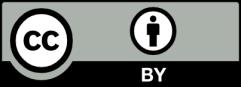
This work is licensed under a Creative Commons Attribution 4.0 International License , which permits unrestricted use, distribution, and reproduction in any medium, provided the original work is properly cited.