Open Access
ARTICLE
Survey on Segmentation and Classification Techniques of Satellite Images by Deep Learning Algorithm
1 Department of Computer Sciences, University of Monastir, Monastir, 1001, Tunisia
2 Research Laboratory in Algebra, Numbers Theory and Intelligent Systems, Monastir, 1001, Tunisia
* Corresponding Author: Atheer Joudah. Email:
Computers, Materials & Continua 2023, 75(3), 4973-4984. https://doi.org/10.32604/cmc.2023.036483
Received 01 October 2022; Accepted 08 February 2023; Issue published 29 April 2023
Abstract
This survey paper aims to show methods to analyze and classify field satellite images using deep learning and machine learning algorithms. Users of deep learning-based Convolutional Neural Network (CNN) technology to harvest fields from satellite images or generate zones of interest were among the planned application scenarios (ROI). Using machine learning, the satellite image is placed on the input image, segmented, and then tagged. In contemporary categorization, field size ratio, Local Binary Pattern (LBP) histograms, and color data are taken into account. Field satellite image localization has several practical applications, including pest management, scene analysis, and field tracking. The relationship between satellite images in a specific area, or contextual information, is essential to comprehending the field in its whole.Keywords
Cite This Article
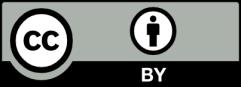
This work is licensed under a Creative Commons Attribution 4.0 International License , which permits unrestricted use, distribution, and reproduction in any medium, provided the original work is properly cited.