Open Access
ARTICLE
Residual Feature Attentional Fusion Network for Lightweight Chest CT Image Super-Resolution
1 College of Quality and Technical Supervision, Hebei University, Baoding, 071002, China
2 Hebei Technology Innovation Center for Lightweight of New Energy Vehicle Power System, Baoding, 071002, China
3 Postdoctoral Research Station of Optical Engineering, Hebei University, Baoding, 071000, China
* Corresponding Author: Kun Liu. Email:
Computers, Materials & Continua 2023, 75(3), 5159-5176. https://doi.org/10.32604/cmc.2023.036401
Received 29 September 2022; Accepted 22 February 2023; Issue published 29 April 2023
Abstract
The diagnosis of COVID-19 requires chest computed tomography (CT). High-resolution CT images can provide more diagnostic information to help doctors better diagnose the disease, so it is of clinical importance to study super-resolution (SR) algorithms applied to CT images to improve the resolution of CT images. However, most of the existing SR algorithms are studied based on natural images, which are not suitable for medical images; and most of these algorithms improve the reconstruction quality by increasing the network depth, which is not suitable for machines with limited resources. To alleviate these issues, we propose a residual feature attentional fusion network for lightweight chest CT image super-resolution (RFAFN). Specifically, we design a contextual feature extraction block (CFEB) that can extract CT image features more efficiently and accurately than ordinary residual blocks. In addition, we propose a feature-weighted cascading strategy (FWCS) based on attentional feature fusion blocks (AFFB) to utilize the high-frequency detail information extracted by CFEB as much as possible via selectively fusing adjacent level feature information. Finally, we suggest a global hierarchical feature fusion strategy (GHFFS), which can utilize the hierarchical features more effectively than dense concatenation by progressively aggregating the feature information at various levels. Numerous experiments show that our method performs better than most of the state-of-the-art (SOTA) methods on the COVID-19 chest CT dataset. In detail, the peak signal-to-noise ratio (PSNR) is 0.11 dB and 0.47 dB higher on CTtest1 and CTtest2 at SR compared to the suboptimal method, but the number of parameters and multi-adds are reduced by 22K and 0.43G, respectively. Our method can better recover chest CT image quality with fewer computational resources and effectively assist in COVID-19.Keywords
Cite This Article
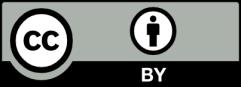