Open Access
ARTICLE
Improved HardNet and Stricter Outlier Filtering to Guide Reliable Matching
1 Queen Mary University of London, London, E14NS, UK
2 Didi Chuxing, Beijing, 100193, China
3 Peking University, Beijing, 100091, China
* Corresponding Author: Pengfei Xu. Email:
Computers, Materials & Continua 2023, 75(3), 4785-4803. https://doi.org/10.32604/cmc.2023.034053
Received 05 July 2022; Accepted 28 September 2022; Issue published 29 April 2023
Abstract
As the fundamental problem in the computer vision area, image matching has wide applications in pose estimation, 3D reconstruction, image retrieval, etc. Suffering from the influence of external factors, the process of image matching using classical local detectors, e.g., scale-invariant feature transform (SIFT), and the outlier filtering approaches, e.g., Random sample consensus (RANSAC), show high computation speed and pool robustness under changing illumination and viewpoints conditions, while image matching approaches with deep learning strategy (such as HardNet, OANet) display reliable achievements in large-scale datasets with challenging scenes. However, the past learning-based approaches are limited to the distinction and quality of the dataset and the training strategy in the image-matching approaches. As an extension of the previous conference paper, this paper proposes an accurate and robust image matching approach using fewer training data in an end-to-end manner, which could be used to estimate the pose error This research first proposes a novel dataset cleaning and construction strategy to eliminate the noise and improve the training efficiency; Secondly, a novel loss named quadratic hinge triplet loss (QHT) is proposed to gather more effective and stable feature matching; Thirdly, in the outlier filtering process, the stricter OANet and bundle adjustment are applied for judging samples by adding the epipolar distance constraint and triangulation constraint to generate more outstanding matches; Finally, to recall the matching pairs, dynamic guided matching is used and then submit the inliers after the PyRANSAC process. Multiple evaluation metrics are used and reported in the 1st place in the Track1 of CVPR Image-Matching Challenge Workshop. The results show that the proposed method has advanced performance in large-scale and challenging Phototourism benchmark.Keywords
Cite This Article
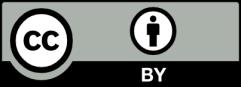
This work is licensed under a Creative Commons Attribution 4.0 International License , which permits unrestricted use, distribution, and reproduction in any medium, provided the original work is properly cited.