Open Access
ARTICLE
Data Augmentation Using Contour Image for Convolutional Neural Network
1 Department of Computer Engineering, Anyang University, Anyang-si, 14058, Korea
2 Department of ICT Convergence Engineering, Anyang University, Anyang-si, 14058, Korea
* Corresponding Author: Jeong-Joon Kim. Email:
Computers, Materials & Continua 2023, 75(3), 4669-4680. https://doi.org/10.32604/cmc.2023.031129
Received 11 April 2022; Accepted 12 June 2022; Issue published 29 April 2023
Abstract
With the development of artificial intelligence-related technologies such as deep learning, various organizations, including the government, are making various efforts to generate and manage big data for use in artificial intelligence. However, it is difficult to acquire big data due to various social problems and restrictions such as personal information leakage. There are many problems in introducing technology in fields that do not have enough training data necessary to apply deep learning technology. Therefore, this study proposes a mixed contour data augmentation technique, which is a data augmentation technique using contour images, to solve a problem caused by a lack of data. ResNet, a famous convolutional neural network (CNN) architecture, and CIFAR-10, a benchmark data set, are used for experimental performance evaluation to prove the superiority of the proposed method. And to prove that high performance improvement can be achieved even with a small training dataset, the ratio of the training dataset was divided into 70%, 50%, and 30% for comparative analysis. As a result of applying the mixed contour data augmentation technique, it was possible to achieve a classification accuracy improvement of up to 4.64% and high accuracy even with a small amount of data set. In addition, it is expected that the mixed contour data augmentation technique can be applied in various fields by proving the excellence of the proposed data augmentation technique using benchmark datasets.Keywords
Cite This Article
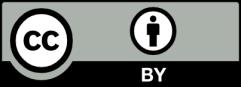
This work is licensed under a Creative Commons Attribution 4.0 International License , which permits unrestricted use, distribution, and reproduction in any medium, provided the original work is properly cited.