Open Access
ARTICLE
Metaheuristic Optimization with Deep Learning Enabled Smart Grid Stability Prediction
Department of Mathematics, College of Science and Humanities in Al-Kharj, Prince Sattam bin Abdulaziz University, Al-Kharj, 11942, Saudi Arabia
* Corresponding Author: Afrah Al-Bossly. Email:
Computers, Materials & Continua 2023, 75(3), 6395-6408. https://doi.org/10.32604/cmc.2023.028433
Received 09 February 2022; Accepted 18 March 2022; Issue published 29 April 2023
Abstract
Due to the drastic increase in global population as well as economy, electricity demand becomes considerably high. The recently developed smart grid (SG) technology has the ability to minimize power loss at the time of power distribution. Machine learning (ML) and deep learning (DL) models can be effectually developed for the design of SG stability techniques. This article introduces a new Social Spider Optimization with Deep Learning Enabled Statistical Analysis for Smart Grid Stability (SSODLSA-SGS) prediction model. Primarily, class imbalance data handling process is performed using Synthetic minority oversampling technique (SMOTE) technique. The SSODLSA-SGS model involves two stages of pre-processing namely data normalization and transformation. Besides, the SSODLSA-SGS model derives a deep belief-back propagation neural network (DBN-BN) model for the prediction of SG stability. Finally, social spider optimization (SSO) algorithm can be applied for determining the optimal hyperparameter values of the DBN-BN model. The design of SSO algorithm helps to appropriately modify the hyperparameter values of the DBN-BN model. A series of simulation analyses are carried out to highlight the enhanced outcomes of the SSODLSA-SGS model. The extensive comparative study reported the enhanced performance of the SSODLSA-SGS algorithm over the other recent techniques interms of several measures.Keywords
Cite This Article
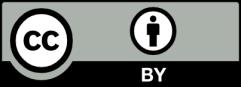
This work is licensed under a Creative Commons Attribution 4.0 International License , which permits unrestricted use, distribution, and reproduction in any medium, provided the original work is properly cited.