Open Access
ARTICLE
Identification of Rice Leaf Disease Using Improved ShuffleNet V2
College of Information and Technology, Jilin Agricultural University, Changchun, 130118, China
* Corresponding Author: Yanlei Xu. Email:
Computers, Materials & Continua 2023, 75(2), 4501-4517. https://doi.org/10.32604/cmc.2023.038446
Received 13 December 2022; Accepted 13 February 2023; Issue published 31 March 2023
Abstract
Accurate identification of rice diseases is crucial for controlling diseases and improving rice yield. To improve the classification accuracy of rice diseases, this paper proposed a classification and identification method based on an improved ShuffleNet V2 (GE-ShuffleNet) model. Firstly, the Ghost module is used to replace the convolution in the two basic unit modules of ShuffleNet V2, and the unimportant convolution is deleted from the two basic unit modules of ShuffleNet V2. The Hardswish activation function is applied to replace the ReLU activation function to improve the identification accuracy of the model. Secondly, an effective channel attention (ECA) module is added to the network to avoid dimension reduction, and the correlation between channels is effectively extracted through 1D convolution. Besides, L2 regularization is introduced to fine-tune the training parameters during training to prevent overfitting. Finally, the considerable experimental and numerical results proved the advantages of our proposed model in terms of model size, floating-point operation per second (FLOPs), and parameters (Params). Especially in the case of smaller model size (5.879 M), the identification accuracy of GE-ShuffleNet (96.6%) is higher than that of ShuffleNet V2 (94.4%), MobileNet V2 (93.7%), AlexNet (79.1%), Swim Transformer (88.1%), EfficientNet V2 (89.7%), VGG16 (81.9%), GhostNet (89.3%) and ResNet50 (92.5%).Keywords
Cite This Article
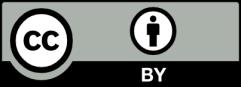