Open Access
ARTICLE
TECMH: Transformer-Based Cross-Modal Hashing For Fine-Grained Image-Text Retrieval
1 College of Computer and Information Science, Chongqing Normal University, Chongqing, 401331, China
2 Institute of Systems and Robotics (ISR), Department of Electrical and Computer Engineering (DEEC),University of Coimbra, Coimbra, Portugal
* Corresponding Author: Mingyong Li. Email:
Computers, Materials & Continua 2023, 75(2), 3713-3728. https://doi.org/10.32604/cmc.2023.037463
Received 04 November 2022; Accepted 30 January 2023; Issue published 31 March 2023
Abstract
In recent years, cross-modal hash retrieval has become a popular research field because of its advantages of high efficiency and low storage. Cross-modal retrieval technology can be applied to search engines, cross-modal medical processing, etc. The existing main method is to use a multi-label matching paradigm to finish the retrieval tasks. However, such methods do not use fine-grained information in the multi-modal data, which may lead to sub-optimal results. To avoid cross-modal matching turning into label matching, this paper proposes an end-to-end fine-grained cross-modal hash retrieval method, which can focus more on the fine-grained semantic information of multi-modal data. First, the method refines the image features and no longer uses multiple labels to represent text features but uses BERT for processing. Second, this method uses the inference capabilities of the transformer encoder to generate global fine-grained features. Finally, in order to better judge the effect of the fine-grained model, this paper uses the datasets in the image text matching field instead of the traditional label-matching datasets. This article experiment on Microsoft COCO (MS-COCO) and Flickr30K datasets and compare it with the previous classical methods. The experimental results show that this method can obtain more advanced results in the cross-modal hash retrieval field.Keywords
Cite This Article
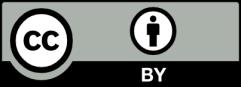