Open Access
ARTICLE
Modified Wild Horse Optimization with Deep Learning Enabled Symmetric Human Activity Recognition Model
1 Accounting Department, College of Administration and Economics, University of Duhok, Duhok, Iraq
2 Computer Science Department, College of Science, Nawroz University, Duhok, Iraq
3 ITM Department, Technical College of Administrative, Duhok Polytechnic University, Duhok, Iraq
4 Energy Eng. Department, Technical College of Engineering, Duhok Polytechnic University, Duhok, Iraq
* Corresponding Author: Subhi R. M. Zeebaree. Email:
Computers, Materials & Continua 2023, 75(2), 4009-4024. https://doi.org/10.32604/cmc.2023.037433
Received 03 November 2022; Accepted 08 February 2023; Issue published 31 March 2023
Abstract
Traditional indoor human activity recognition (HAR) is a time-series data classification problem and needs feature extraction. Presently, considerable attention has been given to the domain of HAR due to the enormous amount of its real-time uses in real-time applications, namely surveillance by authorities, biometric user identification, and health monitoring of older people. The extensive usage of the Internet of Things (IoT) and wearable sensor devices has made the topic of HAR a vital subject in ubiquitous and mobile computing. The more commonly utilized inference and problem-solving technique in the HAR system have recently been deep learning (DL). The study develops a Modified Wild Horse Optimization with DL Aided Symmetric Human Activity Recognition (MWHODL-SHAR) model. The major intention of the MWHODL-SHAR model lies in recognition of symmetric activities, namely jogging, walking, standing, sitting, etc. In the presented MWHODL-SHAR technique, the human activities data is pre-processed in various stages to make it compatible for further processing. A convolution neural network with an attention-based long short-term memory (CNN-ALSTM) model is applied for activity recognition. The MWHO algorithm is utilized as a hyperparameter tuning strategy to improve the detection rate of the CNN-ALSTM algorithm. The experimental validation of the MWHODL-SHAR technique is simulated using a benchmark dataset. An extensive comparison study revealed the betterment of the MWHODL-SHAR technique over other recent approaches.Keywords
Cite This Article
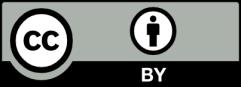
This work is licensed under a Creative Commons Attribution 4.0 International License , which permits unrestricted use, distribution, and reproduction in any medium, provided the original work is properly cited.