Open Access
ARTICLE
COVID-19 Classification from X-Ray Images: An Approach to Implement Federated Learning on Decentralized Dataset
1 Department of Telecommunication Engineering, Sir Syed University of Engineering & Technology, Karachi, 75300, Pakistan
2 Department of Information Technology, College of Computer and Information Sciences, Princess Nourah bint Abdulrahman University, P.O. Box 84428, Riyadh, 11671, Saudi Arabia
3 Security Engineering Lab, Computer Science Department, Prince Sultan University, Riyadh, 11586, Saudi Arabia
4 Department of Electronics and Electrical Communications Engineering, Faculty of Electronic Engineering, Menoufia University, Menouf, 32952, Egypt
* Corresponding Author: Naglaa F. Soliman. Email:
Computers, Materials & Continua 2023, 75(2), 3883-3901. https://doi.org/10.32604/cmc.2023.037413
Received 03 November 2022; Accepted 08 February 2023; Issue published 31 March 2023
Abstract
The COVID-19 pandemic has devastated our daily lives, leaving horrific repercussions in its aftermath. Due to its rapid spread, it was quite difficult for medical personnel to diagnose it in such a big quantity. Patients who test positive for Covid-19 are diagnosed via a nasal PCR test. In comparison, polymerase chain reaction (PCR) findings take a few hours to a few days. The PCR test is expensive, although the government may bear expenses in certain places. Furthermore, subsets of the population resist invasive testing like swabs. Therefore, chest X-rays or Computerized Vomography (CT) scans are preferred in most cases, and more importantly, they are non-invasive, inexpensive, and provide a faster response time. Recent advances in Artificial Intelligence (AI), in combination with state-of-the-art methods, have allowed for the diagnosis of COVID-19 using chest x-rays. This article proposes a method for classifying COVID-19 as positive or negative on a decentralized dataset that is based on the Federated learning scheme. In order to build a progressive global COVID-19 classification model, two edge devices are employed to train the model on their respective localized dataset, and a 3-layered custom Convolutional Neural Network (CNN) model is used in the process of training the model, which can be deployed from the server. These two edge devices then communicate their learned parameter and weight to the server, where it aggregates and updates the global model. The proposed model is trained using an image dataset that can be found on Kaggle. There are more than 13,000 X-ray images in Kaggle Database collection, from that collection 9000 images of Normal and COVID-19 positive images are used. Each edge node possesses a different number of images; edge node 1 has 3200 images, while edge node 2 has 5800. There is no association between the datasets of the various nodes that are included in the network. By doing it in this manner, each of the nodes will have access to a separate image collection that has no correlation with each other. The diagnosis of COVID-19 has become considerably more efficient with the installation of the suggested algorithm and dataset, and the findings that we have obtained are quite encouraging.Keywords
Cite This Article
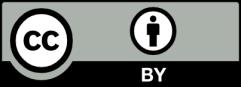
This work is licensed under a Creative Commons Attribution 4.0 International License , which permits unrestricted use, distribution, and reproduction in any medium, provided the original work is properly cited.