Open Access
ARTICLE
Automated Colonic Polyp Detection and Classification Enabled Northern Goshawk Optimization with Deep Learning
1 Engineering College, Al-Kitab University, Kirkuk, Iraq
2 Information System Engineering Department, Erbil Technical Engineering College, Erbil Polytechnic University, Erbil, Iraq
3 Energy Eng. Department, Technical College of Engineering, Duhok Polytechnic University, Duhok, Iraq
4 Computer Science Department, College of Science, Nawroz University, Duhok, Iraq
* Corresponding Author: Subhi R. M. Zeebaree. Email:
Computers, Materials & Continua 2023, 75(2), 3677-3693. https://doi.org/10.32604/cmc.2023.037363
Received 01 November 2022; Accepted 15 January 2023; Issue published 31 March 2023
Abstract
The major mortality factor relevant to the intestinal tract is the growth of tumorous cells (polyps) in various parts. More specifically, colonic polyps have a high rate and are recognized as a precursor of colon cancer growth. Endoscopy is the conventional technique for detecting colon polyps, and considerable research has proved that automated diagnosis of image regions that might have polyps within the colon might be used to help experts for decreasing the polyp miss rate. The automated diagnosis of polyps in a computer-aided diagnosis (CAD) method is implemented using statistical analysis. Nowadays, Deep Learning, particularly through Convolution Neural networks (CNN), is broadly employed to allow the extraction of representative features. This manuscript devises a new Northern Goshawk Optimization with Transfer Learning Model for Colonic Polyp Detection and Classification (NGOTL-CPDC) model. The NGOTL-CPDC technique aims to investigate endoscopic images for automated colonic polyp detection. To accomplish this, the NGOTL-CPDC technique comprises of adaptive bilateral filtering (ABF) technique as a noise removal process and image pre-processing step. Besides, the NGOTL-CPDC model applies the Faster SqueezeNet model for feature extraction purposes in which the hyperparameter tuning process is performed using the NGO optimizer. Finally, the fuzzy Hopfield neural network (FHNN) method can be employed for colonic poly detection and classification. A widespread simulation analysis is carried out to ensure the improved outcomes of the NGOTL-CPDC model. The comparison study demonstrates the enhancements of the NGOTL-CPDC model on the colonic polyp classification process on medical test images.Keywords
Cite This Article
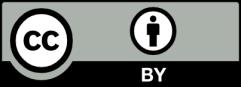
This work is licensed under a Creative Commons Attribution 4.0 International License , which permits unrestricted use, distribution, and reproduction in any medium, provided the original work is properly cited.