Open Access
ARTICLE
Multi-Task Learning Model with Data Augmentation for Arabic Aspect-Based Sentiment Analysis
1 Department of Computer Science, Faculty of Computing and Information Technology, King Abdulaziz University, Jeddah, 21589, Saudi Arabia
2 Department of Computer Science, Faculty of Computer Sciences and Engineering, Hodeidah University, Yemen
* Corresponding Author: Arwa Saif Fadel. Email:
Computers, Materials & Continua 2023, 75(2), 4419-4444. https://doi.org/10.32604/cmc.2023.037112
Received 23 October 2022; Accepted 30 January 2023; Issue published 31 March 2023
Abstract
Aspect-based sentiment analysis (ABSA) is a fine-grained process. Its fundamental subtasks are aspect term extraction (ATE) and aspect polarity classification (APC), and these subtasks are dependent and closely related. However, most existing works on Arabic ABSA content separately address them, assume that aspect terms are preidentified, or use a pipeline model. Pipeline solutions design different models for each task, and the output from the ATE model is used as the input to the APC model, which may result in error propagation among different steps because APC is affected by ATE error. These methods are impractical for real-world scenarios where the ATE task is the base task for APC, and its result impacts the accuracy of APC. Thus, in this study, we focused on a multi-task learning model for Arabic ATE and APC in which the model is jointly trained on two subtasks simultaneously in a single model. This paper integrates the multi-task model, namely Local Cotext Foucse-Aspect Term Extraction and Polarity classification (LCF-ATEPC) and Arabic Bidirectional Encoder Representation from Transformers (AraBERT) as a shred layer for Arabic contextual text representation. The LCF-ATEPC model is based on a multi-head self-attention and local context focus mechanism (LCF) to capture the interactive information between an aspect and its context. Moreover, data augmentation techniques are proposed based on state-of-the-art augmentation techniques (word embedding substitution with constraints and contextual embedding (AraBERT)) to increase the diversity of the training dataset. This paper examined the effect of data augmentation on the multi-task model for Arabic ABSA. Extensive experiments were conducted on the original and combined datasets (merging the original and augmented datasets). Experimental results demonstrate that the proposed Multi-task model outperformed existing APC techniques. Superior results were obtained by AraBERT and LCF-ATEPC with fusion layer (AR-LCF-ATEPC-Fusion) and the proposed data augmentation word embedding-based method (FastText) on the combined dataset.Keywords
Cite This Article
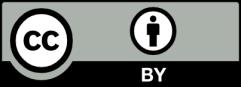