Open Access
ARTICLE
Syntax-Based Aspect Sentiment Quad Prediction by Dual Modules Neural Network for Chinese Comments
1 College of Information and Communication Engineering, Communication University of China, Dingfuzhuang East Street No. 1, Beijing, 100024, China
2 Cybersecurity and Criminology Centre, University of West London, St. Mary’s Road, London W5 5RF, London, UK
3 Department of Electronic Engineering, Tsinghua University, Shuangqing Road No. 30, Beijing, 100084, China
4 The State Key Laboratory of Media Convergence and Communication, Communication University of China, Dingfuzhuang East Street No. 1, Beijing, 100024, China
* Corresponding Author: Fulian Yin. Email:
Computers, Materials & Continua 2023, 75(2), 2873-2888. https://doi.org/10.32604/cmc.2023.037060
Received 21 October 2022; Accepted 06 January 2023; Issue published 31 March 2023
Abstract
Aspect-Based Sentiment Analysis (ABSA) is one of the essential research in the field of Natural Language Processing (NLP), of which Aspect Sentiment Quad Prediction (ASQP) is a novel and complete subtask. ASQP aims to accurately recognize the sentiment quad in the target sentence, which includes the aspect term, the aspect category, the corresponding opinion term, and the sentiment polarity of opinion. Nevertheless, existing approaches lack knowledge of the sentence’s syntax, so despite recent innovations in ASQP, it is poor for complex cyber comment processing. Also, most research has focused on processing English text, and ASQP for Chinese text is almost non-existent. Chinese usage is more casual than English, and individual characters contain more information. We propose a novel syntactically enhanced neural network framework inspired by syntax knowledge enhancement strategies in other NLP studies. In this framework, part of speech (POS) and dependency trees are input to the model as auxiliary information to strengthen its cognition of Chinese text structure. Besides, we design a relation extraction module, which provides a bridge for the overall extraction of the framework. A comparison of the designed experiments reveals that our proposed strategy outperforms the previous studies on the key metric F1. Further experiments demonstrate that the auxiliary information added to the framework improves the final performance in different ways.Keywords
Cite This Article
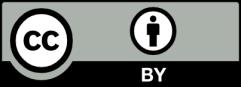
This work is licensed under a Creative Commons Attribution 4.0 International License , which permits unrestricted use, distribution, and reproduction in any medium, provided the original work is properly cited.