Open Access
ARTICLE
Image Emotion Classification Network Based on Multilayer Attentional Interaction, Adaptive Feature Aggregation
1 Engineering Research Center of Digital Forensics, Ministry of Education, Jiangsu Engineering Center of Network Monitoring, School of Computer and Software, Nanjing University of Information Science & Technology, Nanjing, 210044, China
2 Wuxi Research Institute, Nanjing University of Information Science & Technology, Wuxi, 214100, China
3 Jiangsu Collaborative Innovation Center of Atmospheric Environment and Equipment Technology (CICAEET), Nanjing University of Information Science & Technology, Nanjing, 210044, China
4 School of Automation, Nanjing University of Information Science & Technology, Nanjing, 210044, China
5 Adani University, Ahmedabad, Gujarat, India
* Corresponding Author: Xiaorui Zhang. Email:
Computers, Materials & Continua 2023, 75(2), 4273-4291. https://doi.org/10.32604/cmc.2023.036975
Received 18 October 2022; Accepted 30 January 2023; Issue published 31 March 2023
Abstract
The image emotion classification task aims to use the model to automatically predict the emotional response of people when they see the image. Studies have shown that certain local regions are more likely to inspire an emotional response than the whole image. However, existing methods perform poorly in predicting the details of emotional regions and are prone to overfitting during training due to the small size of the dataset. Therefore, this study proposes an image emotion classification network based on multilayer attentional interaction and adaptive feature aggregation. To perform more accurate emotional region prediction, this study designs a multilayer attentional interaction module. The module calculates spatial attention maps for higher-layer semantic features and fusion features through a multilayer shuffle attention module. Through layer-by-layer up-sampling and gating operations, the higher-layer features guide the lower-layer features to learn, eventually achieving sentiment region prediction at the optimal scale. To complement the important information lost by layer-by-layer fusion, this study not only adds an intra-layer fusion to the multilayer attention interaction module but also designs an adaptive feature aggregation module. The module uses global average pooling to compress spatial information and connect channel information from all layers. Then, the module adaptively generates a set of aggregated weights through two fully connected layers to augment the original features of each layer. Eventually, the semantics and details of the different layers are aggregated through gating operations and residual connectivity to complement the lost information. To reduce overfitting on small datasets, the network is pre-trained on the FI dataset, and further weight fine-tuning is performed on the small dataset. The experimental results on the FI, Twitter I and Emotion ROI (Region of Interest) datasets show that the proposed network exceeds existing image emotion classification methods, with accuracies of 90.27%, 84.66% and 84.96%.Keywords
Cite This Article
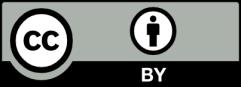