Open Access
ARTICLE
Optimal Wavelet Neural Network-Based Intrusion Detection in Internet of Things Environment
1 Department of Electrical Engineering, College of Engineering, Princess Nourah bint Abdulrahman University, P.O. Box, 84428, Riyadh 11671, Saudi Arabia
2 Department of Computer Sciences, College of Computer and Information Sciences, Princess Nourah bint Abdulrahman University, P.O. Box, 84428, Riyadh 11671, Saudi Arabia
3 Department of Computer Science, College of Computer, Qassim University, Saudi Arabia
4 Department of Computer Science, College of Computer Engineering and Sciences, Prince Sattam bin Abdulaziz University, Al-Kharj, 16273, Saudi Arabia
5 Department of Computer and Self Development, Preparatory Year Deanship, Prince Sattam bin Abdulaziz University, AlKharj, Saudi Arabia
* Corresponding Author: Mesfer Al Duhayyim. Email:
Computers, Materials & Continua 2023, 75(2), 4467-4483. https://doi.org/10.32604/cmc.2023.036822
Received 13 October 2022; Accepted 17 February 2023; Issue published 31 March 2023
Abstract
As the Internet of Things (IoT) endures to develop, a huge count of data has been created. An IoT platform is rather sensitive to security challenges as individual data can be leaked, or sensor data could be used to cause accidents. As typical intrusion detection system (IDS) studies can be frequently designed for working well on databases, it can be unknown if they intend to work well in altering network environments. Machine learning (ML) techniques are depicted to have a higher capacity at assisting mitigate an attack on IoT device and another edge system with reasonable accuracy. This article introduces a new Bird Swarm Algorithm with Wavelet Neural Network for Intrusion Detection (BSAWNN-ID) in the IoT platform. The main intention of the BSAWNN-ID algorithm lies in detecting and classifying intrusions in the IoT platform. The BSAWNN-ID technique primarily designs a feature subset selection using the coyote optimization algorithm (FSS-COA) to attain this. Next, to detect intrusions, the WNN model is utilized. At last, the WNN parameters are optimally modified by the use of BSA. A widespread experiment is performed to depict the better performance of the BSAWNN-ID technique. The resultant values indicated the better performance of the BSAWNN-ID technique over other models, with an accuracy of 99.64% on the UNSW-NB15 dataset.Keywords
Cite This Article
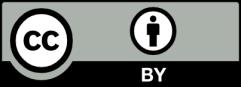
This work is licensed under a Creative Commons Attribution 4.0 International License , which permits unrestricted use, distribution, and reproduction in any medium, provided the original work is properly cited.