Open Access
ARTICLE
An Efficient Text-Independent Speaker Identification Using Feature Fusion and Transformer Model
1 College of Computing, Khon Kaen University, Khon Kaen, 40000, Thailand
2 Department of Computer Science, COMSATS University Islamabad, Vehari Campus, Vehari, 61100, Pakistan
3 Department of Computer Science, College of Computers and Information Technology, Taif University, P.O. Box 11099, Taif, 21944, Saudi Arabia
4 Department of Computer Science, Community College in Dwadmi, Sharqa University, Dawadmi, 17472, Saudi Arabia
5 School of Telecommunication Engineering, Suranaree University of Technology, Nakhon Ratchasima, 30000, Thailand
* Corresponding Author: Rashid Jahangir. Email:
Computers, Materials & Continua 2023, 75(2), 4085-4100. https://doi.org/10.32604/cmc.2023.036797
Received 12 October 2022; Accepted 30 January 2023; Issue published 31 March 2023
Abstract
Automatic Speaker Identification (ASI) involves the process of distinguishing an audio stream associated with numerous speakers’ utterances. Some common aspects, such as the framework difference, overlapping of different sound events, and the presence of various sound sources during recording, make the ASI task much more complicated and complex. This research proposes a deep learning model to improve the accuracy of the ASI system and reduce the model training time under limited computation resources. In this research, the performance of the transformer model is investigated. Seven audio features, chromagram, Mel-spectrogram, tonnetz, Mel-Frequency Cepstral Coefficients (MFCCs), delta MFCCs, delta-delta MFCCs and spectral contrast, are extracted from the ELSDSR, CSTR-VCTK, and Ar-DAD, datasets. The evaluation of various experiments demonstrates that the best performance was achieved by the proposed transformer model using seven audio features on all datasets. For ELSDSR, CSTR-VCTK, and Ar-DAD, the highest attained accuracies are 0.99, 0.97, and 0.99, respectively. The experimental results reveal that the proposed technique can achieve the best performance for ASI problems.Keywords
Cite This Article
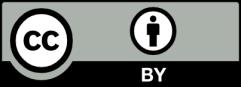
This work is licensed under a Creative Commons Attribution 4.0 International License , which permits unrestricted use, distribution, and reproduction in any medium, provided the original work is properly cited.