Open Access
ARTICLE
Reinforcing Artificial Neural Networks through Traditional Machine Learning Algorithms for Robust Classification of Cancer
1 Department of Computer Sciences and Information Technology, University of Azad Jammu and Kashmir, Muzaffarabad, 13100, Pakistan
2 Department of Computer Science and AI, College of Computer Science and Engineering, University of Jeddah, Jeddah, 23890, Saudi Arabia
3 Department of Computer and Network Engineering, College of Computer Science and Engineering, University of Jeddah, Jeddah, 23890, Saudi Arabia
4 Department of AI & DS, School of Computing, National University of Computer and Emerging Sciences, Islamabad, 44000, Pakistan
* Corresponding Author: Malik Sajjad Ahmed Nadeem. Email:
Computers, Materials & Continua 2023, 75(2), 4293-4315. https://doi.org/10.32604/cmc.2023.036710
Received 10 October 2022; Accepted 30 January 2023; Issue published 31 March 2023
Abstract
Machine Learning (ML)-based prediction and classification systems employ data and learning algorithms to forecast target values. However, improving predictive accuracy is a crucial step for informed decision-making. In the healthcare domain, data are available in the form of genetic profiles and clinical characteristics to build prediction models for complex tasks like cancer detection or diagnosis. Among ML algorithms, Artificial Neural Networks (ANNs) are considered the most suitable framework for many classification tasks. The network weights and the activation functions are the two crucial elements in the learning process of an ANN. These weights affect the prediction ability and the convergence efficiency of the network. In traditional settings, ANNs assign random weights to the inputs. This research aims to develop a learning system for reliable cancer prediction by initializing more realistic weights computed using a supervised setting instead of random weights. The proposed learning system uses hybrid and traditional machine learning techniques such as Support Vector Machine (SVM), Linear Discriminant Analysis (LDA), Random Forest (RF), k-Nearest Neighbour (kNN), and ANN to achieve better accuracy in colon and breast cancer classification. This system computes the confusion matrix-based metrics for traditional and proposed frameworks. The proposed framework attains the highest accuracy of 89.24 percent using the colon cancer dataset and 72.20 percent using the breast cancer dataset, which outperforms the other models. The results show that the proposed learning system has higher predictive accuracies than conventional classifiers for each dataset, overcoming previous research limitations. Moreover, the proposed framework is of use to predict and classify cancer patients accurately. Consequently, this will facilitate the effective management of cancer patients.Keywords
Cite This Article
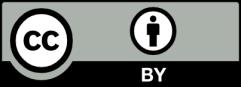
This work is licensed under a Creative Commons Attribution 4.0 International License , which permits unrestricted use, distribution, and reproduction in any medium, provided the original work is properly cited.