Open Access
ARTICLE
Type 2 Diabetes Risk Prediction Using Deep Convolutional Neural Network Based-Bayesian Optimization
1 Computer & Information Sciences, Universiti Teknologi PETRONAS, Seri Iskandar, 32610, Perak, Malaysia
2 Centre for Research in Data Science (CERDAS), Universiti Teknologi PETRONAS, Seri Iskandar, 32610, Perak, Malaysia
3 Department of Imaging Physics, University of Texas MD Anderson Cancer, Houston, TX, USA
* Corresponding Author: Alawi Alqushaibi. Email:
Computers, Materials & Continua 2023, 75(2), 3223-3238. https://doi.org/10.32604/cmc.2023.035655
Received 29 August 2022; Accepted 08 December 2022; Issue published 31 March 2023
Abstract
Diabetes mellitus is a long-term condition characterized by hyperglycemia. It could lead to plenty of difficulties. According to rising morbidity in recent years, the world’s diabetic patients will exceed 642 million by 2040, implying that one out of every ten persons will be diabetic. There is no doubt that this startling figure requires immediate attention from industry and academia to promote innovation and growth in diabetes risk prediction to save individuals’ lives. Due to its rapid development, deep learning (DL) was used to predict numerous diseases. However, DL methods still suffer from their limited prediction performance due to the hyperparameters selection and parameters optimization. Therefore, the selection of hyper-parameters is critical in improving classification performance. This study presents Convolutional Neural Network (CNN) that has achieved remarkable results in many medical domains where the Bayesian optimization algorithm (BOA) has been employed for hyperparameters selection and parameters optimization. Two issues have been investigated and solved during the experiment to enhance the results. The first is the dataset class imbalance, which is solved using Synthetic Minority Oversampling Technique (SMOTE) technique. The second issue is the model's poor performance, which has been solved using the Bayesian optimization algorithm. The findings indicate that the Bayesian based-CNN model superbases all the state-of-the-art models in the literature with an accuracy of 89.36%, F1-score of 0.88.6, and Matthews Correlation Coefficient (MCC) of 0.88.6.Keywords
Cite This Article
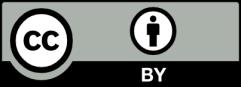
This work is licensed under a Creative Commons Attribution 4.0 International License , which permits unrestricted use, distribution, and reproduction in any medium, provided the original work is properly cited.