Open Access
ARTICLE
A Convolutional Neural Network Model for Wheat Crop Disease Prediction
1 Department of Computer Science and Artificial Intelligence, College of Computer Science and Engineering, University of Jeddah, Jeddah, 21577, Saudi Arabia
2 Department of Computer Science, Bacha Khan University Charsadda, Khyber Pakhtunkhwa, 24461, Pakistan
3 Department of Computer Science, Federal Urdu University of Arts, Science & Technology, Islamabad, 45570, Pakistan
4 Department of Information Systems and Technology, College of Computer Science and Engineering, University of Jeddah, Jeddah, 21577, Saudi Arabia
5 Faculty of Technology and Applied Sciences, Al-Quds Open University, Al-Bireh, 1804, Palestine
6 Department of Computer Science, HITEC University, Taxila, 47080, Pakistan
* Corresponding Author: Mahmood Ashraf. Email:
Computers, Materials & Continua 2023, 75(2), 3867-3882. https://doi.org/10.32604/cmc.2023.035498
Received 23 August 2022; Accepted 08 February 2023; Issue published 31 March 2023
Abstract
Wheat is the most important cereal crop, and its low production incurs import pressure on the economy. It fulfills a significant portion of the daily energy requirements of the human body. The wheat disease is one of the major factors that result in low production and negatively affects the national economy. Thus, timely detection of wheat diseases is necessary for improving production. The CNN-based architectures showed tremendous achievement in the image-based classification and prediction of crop diseases. However, these models are computationally expensive and need a large amount of training data. In this research, a light weighted modified CNN architecture is proposed that uses eight layers particularly, three convolutional layers, three SoftMax layers, and two flattened layers, to detect wheat diseases effectively. The high-resolution images were collected from the fields in Azad Kashmir (Pakistan) and manually annotated by three human experts. The convolutional layers use 16, 32, and 64 filters. Every filter uses a 3 × 3 kernel size. The strides for all convolutional layers are set to 1. In this research, three different variants of datasets are used. These variants S1-70%:15%:15%, S2-75%:15%:10%, and S3-80%:10%:10% (train: validation: test) are used to evaluate the performance of the proposed model. The extensive experiments revealed that the S3 performed better than S1 and S2 datasets with 93% accuracy. The experiment also concludes that a more extensive training set with high-resolution images can detect wheat diseases more accurately.Keywords
Cite This Article
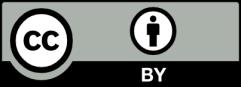