Open Access
ARTICLE
Hybrid Deep Learning Enabled Load Prediction for Energy Storage Systems
1 Department of Mathematics, College of Education, Al-Zahraa University for Women, Karbala, Iraq
2 Biomedical Engineering Department, College of Engineering, University of Warith Al-Anbiyaa, Karbala, Iraq
3 ITM Department, Technical College of Administration, Duhok Polytechnic University, Duhok, Iraq
4 College of Technical Engineering, The Islamic University, Najaf, Iraq
5 Department of Medical Instrumentation Techniques Engineering, Al-Mustaqbal University College, Hillah, 51001, Iraq
6 Computer Technology Engineering, College of Engineering Technology, Al-Kitab University, Kirkuk, 36013, Iraq
7 College of Information Technology, Imam Ja’afar Al-Sadiq University, Al-Muthanna, 66002, Iraq
8 Altoosi University College, Najaf, Iraq
9 Department of Medical Instruments Engineering Techniques, Al-Farahidi University, Bagdad, Iraq
10 Department of Medical Instruments Engineering Techniques, Al-Turath University College, Baghdad, 10021, Iraq
11 Department of Medical Instruments Engineering Techniques, Al-Farahidi University, Baghdad, 10021, Iraq
12 Department of Medical Instrumentations Techniques Engineering, Dijlah University College, Baghdad, Iraq
* Corresponding Author: Ahmed Alkhayyat. Email:
Computers, Materials & Continua 2023, 75(2), 3359-3374. https://doi.org/10.32604/cmc.2023.034221
Received 10 July 2022; Accepted 27 August 2022; Issue published 31 March 2023
Abstract
Recent economic growth and development have considerably raised energy consumption over the globe. Electric load prediction approaches become essential for effective planning, decision-making, and contract evaluation of the power systems. In order to achieve effective forecasting outcomes with minimum computation time, this study develops an improved whale optimization with deep learning enabled load prediction (IWO-DLELP) scheme for energy storage systems (ESS) in smart grid platform. The major intention of the IWO-DLELP technique is to effectually forecast the electric load in SG environment for designing proficient ESS. The proposed IWO-DLELP model initially undergoes pre-processing in two stages namely min-max normalization and feature selection. Besides, partition clustering approach is applied for the decomposition of data into distinct clusters with respect to distance and objective functions. Moreover, IWO with bidirectional gated recurrent unit (BiGRU) model is applied for the prediction of load and the hyperparameters are tuned by the use of IWO algorithm. The experiment analysis reported the enhanced results of the IWO-DLELP model over the recent methods interms of distinct evaluation measures.Keywords
Cite This Article
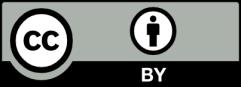
This work is licensed under a Creative Commons Attribution 4.0 International License , which permits unrestricted use, distribution, and reproduction in any medium, provided the original work is properly cited.