Open Access
ARTICLE
Quantum Particle Swarm Optimization with Deep Learning-Based Arabic Tweets Sentiment Analysis
1 Department of Language Preparation, Arabic Language Teaching Institute, Princess Nourah bint Abdulrahman University, P.O. Box 84428, Riyadh, 11671, Saudi Arabia
2 Department of English, College of Science and Arts at Mahayil, King Khalid University, Abha, 62217, Saudi Arabia
3 Department of Computer Sciences, College of Computing and Information System, Umm Al-Qura University, Makkah, 24231, Saudi Arabia
4 Department of Computer Science, College of Sciences and Humanities-Aflaj, Prince Sattam bin Abdulaziz University, Al-Aflaj, 16828, Saudi Arabia
5 Research Centre, Future University in Egypt, New Cairo, 11845, Egypt
6 Department of Computer and Self Development, Preparatory Year Deanship, Prince Sattam bin Abdulaziz University, AlKharj, 16436, Saudi Arabia
* Corresponding Author: Mesfer Al Duhayyim. Email:
Computers, Materials & Continua 2023, 75(2), 2575-2591. https://doi.org/10.32604/cmc.2023.033531
Received 19 June 2022; Accepted 13 October 2022; Issue published 31 March 2023
Abstract
Sentiment Analysis (SA), a Machine Learning (ML) technique, is often applied in the literature. The SA technique is specifically applied to the data collected from social media sites. The research studies conducted earlier upon the SA of the tweets were mostly aimed at automating the feature extraction process. In this background, the current study introduces a novel method called Quantum Particle Swarm Optimization with Deep Learning-Based Sentiment Analysis on Arabic Tweets (QPSODL-SAAT). The presented QPSODL-SAAT model determines and classifies the sentiments of the tweets written in Arabic. Initially, the data pre-processing is performed to convert the raw tweets into a useful format. Then, the word2vec model is applied to generate the feature vectors. The Bidirectional Gated Recurrent Unit (BiGRU) classifier is utilized to identify and classify the sentiments. Finally, the QPSO algorithm is exploited for the optimal fine-tuning of the hyperparameters involved in the BiGRU model. The proposed QPSODL-SAAT model was experimentally validated using the standard datasets. An extensive comparative analysis was conducted, and the proposed model achieved a maximum accuracy of 98.35%. The outcomes confirmed the supremacy of the proposed QPSODL-SAAT model over the rest of the approaches, such as the Surface Features (SF), Generic Embeddings (GE), Arabic Sentiment Embeddings constructed using the Hybrid (ASEH) model and the Bidirectional Encoder Representations from Transformers (BERT) model.Keywords
Cite This Article
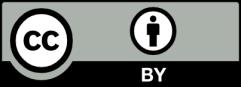
This work is licensed under a Creative Commons Attribution 4.0 International License , which permits unrestricted use, distribution, and reproduction in any medium, provided the original work is properly cited.