Open Access
ARTICLE
Quantum Fuzzy Regression Model for Uncertain Environment
1 School of Cybersecurity, Chengdu University of Information Technology, Chengdu, 610225, China
2 Advanced Cryptography and System Security Key Laboratory of Sichuan Province, Chengdu, 610255, China
3 School of Engineering and Technology, University of Hertfordshire, Hertford, UK
* Corresponding Author: Yan Chang. Email:
Computers, Materials & Continua 2023, 75(2), 2759-2773. https://doi.org/10.32604/cmc.2023.033284
Received 13 June 2022; Accepted 17 November 2022; Issue published 31 March 2023
Abstract
In the era of big data, traditional regression models cannot deal with uncertain big data efficiently and accurately. In order to make up for this deficiency, this paper proposes a quantum fuzzy regression model, which uses fuzzy theory to describe the uncertainty in big data sets and uses quantum computing to exponentially improve the efficiency of data set preprocessing and parameter estimation. In this paper, data envelopment analysis (DEA) is used to calculate the degree of importance of each data point. Meanwhile, Harrow, Hassidim and Lloyd (HHL) algorithm and quantum swap circuits are used to improve the efficiency of high-dimensional data matrix calculation. The application of the quantum fuzzy regression model to small-scale financial data proves that its accuracy is greatly improved compared with the quantum regression model. Moreover, due to the introduction of quantum computing, the speed of dealing with high-dimensional data matrix has an exponential improvement compared with the fuzzy regression model. The quantum fuzzy regression model proposed in this paper combines the advantages of fuzzy theory and quantum computing which can efficiently calculate high-dimensional data matrix and complete parameter estimation using quantum computing while retaining the uncertainty in big data. Thus, it is a new model for efficient and accurate big data processing in uncertain environments.Keywords
Cite This Article
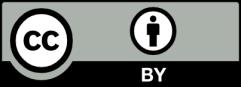