Open Access
ARTICLE
Power Optimized Multiple-UAV Error-Free Network in Cognitive Environment
1 School of Electronics and Electrical Engineering, Lovely Professional University, Phagwara, Punjab, 144411, India
2 Department of Computer Engineering, College of Computer and Information Sciences, Majmaah University, Al-Majmaah, 11952, Saudi Arabia
3 University Centre for Research and Development, Chandigarh University, Mohali, Punjab, 140413, India
4 Department of Computer Science, College of Computer and Information Sciences, Majmaah University, Al-Majmaah, 11952, Saudi Arabia
* Corresponding Author: Ahmed Alhussen. Email:
Computers, Materials & Continua 2023, 75(2), 3189-3201. https://doi.org/10.32604/cmc.2023.030061
Received 17 March 2022; Accepted 31 May 2022; Issue published 31 March 2023
Abstract
Many extensive UAV communication networks have used UAV cooperative control. Wireless networking services can be offered using unmanned aerial vehicles (UAVs) as aerial base stations. Not only is coverage maximization, but also better connectivity, a fundamental design challenge that must be solved. The number of applications for unmanned aerial vehicles (UAVs) operating in unlicensed bands is fast expanding as the Internet of Things (IoT) develops. Those bands, however, have become overcrowded as the number of systems that use them grows. Cognitive Radio (CR) and spectrum allocation approaches have emerged as a potential approach for resolving spectrum scarcity in wireless networks, and hence as technological solutions for future generations, from this perspective. As a result, combining CR with UAVs has the potential to give significant benefits for large-scale UAV deployment. The paper examines existing research on the subject of UAV covering and proposes a multi-UAV cognitive-based error-free model for energy-efficient communication. Coverage maximization, power control, and enhanced connection quality are the three steps of the proposed model. To satisfy the desired signal-to-noise ratio, the covering zone efficacy is investigated as a function of the distance among UAVs stationed in a specific geographic region depending on multiple deployment configurations like as rural, suburban, and urban macro deployment scenarios of the ITU-R M.2135 standard (SNR).Keywords
Wireless communication is a reliable system that makes it easier for people to communicate. To link the computer systems and mobile nodes, there is no need for any established infrastructure with this technique. This technological revolution has resulted in significant advancements in computers and communication, as well as the availability of promised services at any time and from any location. In comparison to wired networks, these are low-cost networks. It provides versatile mobility, allowing users to access information at any time and from any location without relying on a fixed network link [1,2]. The wireless network allows users and nodes to connect securely with one another. Second wireless data use is growing at a higher pace in the next wave of wireless communication. However, the frequency range that can be used is limited and costly. As a result, modern wireless networks must function efficiently to satisfy the increasing demand for wireless services. As a result, mathematical optimization approaches, such are multi-objective.
Optimization tools and traditional optimization methods play a critical role in improving the performance of wireless networks. The communication network for unmanned aerial vehicles has been the subject of this paper. The UAVs are self-contained and can fly freely or under the guidance of ground stations. These decentralized nodes’ applications deliver exciting new ways to complete a project, whether it’s a government or industrial mission. A single UAV design is currently in development. The Cloud of UAVs, on the other hand, has several advantages. As a result, there are several providing operational and consulting services in UAVCN [3]. Even so, independent UAVs often tend to meet the requirements of complex missions, limiting their use in a wide range of applications. To solve the problem, a collective UAV group must be formed to improve performance, particularly in data collection and processing. The authors, for example, developed a model of inter cooperative investigation [4–8]. A collaborative exploration project focused on object tracking, recognition, and latency was investigated in this study. Aside from covering capacity, there are indeed numerous issues comprising mobility, longevity, compatibility, and obstructions. Energy deficiency is a major flaw in UAVs since it can lead to mission failure or even a drone crash if the power can’t keep up with the drones’ operation completion. As a result, energy-efficient UAV contact in UAV networks must be thoroughly investigated [9]. When UAVs perform purpose surveillance, they can significantly increase their ability to adjust to the environment while lowering contact and flight overhead, avoiding serious consequences. It should be noted that due to the collaborative effect of related UAVs, the UAV reconnaissance mission can be accomplished. Multi-UAV cooperating surveillance, on the other hand, is rarely explored because of the challenge to explain the collaborative relationship. The issue of UAV coverage has been researched. The authors developed a paradigm that reflects the UAV’s energy usage and communication performance to examine energy-efficient UAV interaction with a sensing element. Among the several historical optimization approaches, In the current wireless generation, convergent efficiency has proven to be the best technique for algorithm creation. As a result, it has become the most common engineering tool among researchers worldwide [10,11]. As a result, the convex optimization method can be used to optimize the power. The increased capacity requirements of wireless technologies can only be fulfilled by enormous network densification through the deployment of UAVs. However, the effectiveness of this deployment principle is contingent on an effective deployment strategy [12–17]. Previously, the implementation of macro base stations was primarily focused on improving coverage. This is why researchers all over the world are working to find coverage gaps, or areas that aren’t covered. The deployment of UAVs paved the way not just for increased coverage, but also for increased capacity and power optimization. The proposed method achieves the Inter energy-efficient deployment scenario based on cognitive sensing, which can effectively explore the optimum elucidation. Meanwhile, the projected model convergence was demonstrated. It’s worth noting that our research borrows the idea of coverage probability from and applies it to modeling. The major distinctions can be summarized as follows: Arbitrary installation— is the most basic cognitive-based UAV deployment strategy, in which UAVs are deployed at random.
Edge planned deployment—this strategy solves the issue of network coverage by deploying UAVs in the macro segment without accounting for shadow fading and merely estimating route loss. The deployment of the farthest clusters—this approach can be used to deploy the farthest cluster center by creating the area of the cluster per macrocell. A UAV is then placed in the center of each cluster farthest from its macro BS. The remaining UAVs are distributed at random if the number of UAVs increases with the number of clusters [11]. The aforementioned distribution model is tough to comprehend, achieving certain goals while ignoring others. A deployment model should be covering the full geographical area with the greatest number of users while causing the least amount of interruption and using the least amount of power. In the following section, a new mathematical method is proposed for determining the best locations for UAV deployment for power optimization [13]. In the next section rather than employing a specific criterion for determining whether or not coverage is effective, aggregating the coverage probability of numerous linked UAVs, altering the coverage probability function, which improves the precision and efficiency of UAV coverage. The article considers how transmission power control and position adjustment can improve the energy efficiency of a given number of UAVs [14–16]. A new real-time small object identification (RSOD) method based on the feature expression ability of convolutional networks. To deal with the high-density challenge, the RSOD approach uses shallow feature maps. The Flying Ad Hoc Network is one of the emerging fields that evolved from Flying Ad Hoc Networks (FANET). In any network, a routing protocol has a substantial problem selecting the best optimal path. A multi-feature learning model with enhanced local attention (MFELA) is proposed for vehicle re-identification. The model has both global and local branches. The global branch takes advantage of both middle and high-level semantic features to boost global representation capability [17].
A. Contribution
The paper’s key contribution is in the form of a cooperative network of multi-UAV systems with base station interconnection, which will aid society in the next generation in cognitive radio scenarios by increasing the system’s QoS parameters in terms of FER. The impact of the suggested block diagram on diverse deployment configurations of the ITU-R M.2135 standard, such as urban, suburban, and rural macro deployment setups, is also demonstrated in this study.
B. Organization
The remainder of the paper is laid out as follows. The system model is presented in Section 2, which also introduces the concept of probability of coverage. Section 3 discusses the organized multi-UAV network and its system model, while Section 4 wraps up the paper and suggests some future research topics.
2 System Model and Problem Formulation
Methods for deploying unmanned aerial vehicles (UAVs) to reduce power consumption are discussed as follows.
Consider the case of multi-UAV collaboration. As shown in Fig. 1, there is a coverage problem. For this reason, assume the coverage of the ground unit m. If the likelihood of a given UAV n is Qn, m, we use a term called “soft coverage” to describe our approach. Calculate the chance of m’s being covered by a UAV, and come up with the following formula, shown in Eq. (1).
P=1−(1−Q1,m)(1−Q2,m)(1−Q3,m)…(1−Qn,m)(1)
Figure 1: Scenario of several interfering UAVs
According to this formula, the more UAVs identified, the greater the possibility of coverage for the “m” ground unit, which is both fair and realistic.
A subject worth exploring is how to appropriately characterize a mission point’s coverage utility given the factors of signal power and propagation loss. Consider an air-to-ground network made up of an “N” number of unmanned aerial vehicles (UAVs) [12].
I∈R2 is a continuous region that is meshed uniformly and separated into discrete cells. The state of UAV n is Sn={QnPn} with “Qn” and “Pn” representing UAV n’s horizontal position [[XnYn]] and carrier transmission capacity, respectively. The directional antenna beamwidth of a UAV is denoted by ∅=2π√N0, this corresponds to the antenna layout in a planar array with homogeneous antennas, and the antenna gain of a UAV is denoted by Eq. (2).
G={Gm −ϕ2≤θ≤ϕ2,1sin2(3π/2√N0, otherwise(2)
where Gm denotes main-lobe gain and N0 denotes the number of antenna components. Furthermore, the side lobe benefit is the second item. The coverage probability is affected by propagation loss, position, carrier communication bandwidth, and the ecological impact factor. To begin, for air-to-ground transmission, the route loss L dB is computed by Eq. (3).
LdB=10.n0log(4πfcdinC)(3)
where n0 stands for the path loss exponent, fc is the carrier frequency of the UAV, c is the speed of an electromagnetic wave, and d is the distance between the UAV and mission cell I Second.
Second, there are two types of links: LOS (line-of-sight) and NLOS (non-line-of-sight) due to the faulty wireless transmission. The chance of LOS is calculated by Eq. (4).
PLOS,n=α(180π∅in− ∅0)γ(4)
at which ∅0 is the reflection threshold, which indicates the lowest elevation angle at which a UAV can receive a signal from the LAP. Furthermore, α and γ are environmental effect factors that are constants. As a result, the chance of NLOS is given by Eq. (5).
PNLOS,n=1− PLOS,n(5)
As a result, it is possible to estimate the likelihood of a single UAV out of “n” covering a mission cell “I”, given by Eq. (6).
Qi(Sn)=PLOS,nQ(Pmin+LdB−Pn−G+μLOSσLOS)+PNLOS,nQ(Pmin+LdB−Pn−G+μLOSσLOS)(6)
where Pn is the carrier communication power of UAV n, Pmin denotes the minimum power required for successful detection, and μLOS and σLOS indicate the mean and variance of shadowing for LOS connections, respectively.
This paper presents the notion of mission area importance to evaluate the overall covering consequence of the entire assigned area. As a result, the current UAV network’s capacity to cover assigned cell “I” is limited, refer to Eqs. (7) and (8).
gi,n=σi{1−∏n∈N(1− Qi(Sn))}(7)
where I denote the relevance (traffic, etc.) of mission cell i. As a result, we may determine the coverage capability of the entire UAV network.
U0=∫i∈Igi,N di=∫i∈Iσi{1−∏n∈N(1− Qi(Sn))}di(8)
Because UAVs use their carrier transmission powers to accomplish coverage tasks, reducing energy consumption is a major challenge. On the other side, to assure mission execution excellence, the coverage condition must be met. The UAV setup’s overall energy efficiency G0 is calculated based on the energy efficiency design of UAV communication, shown in Eq. (9).
G0={U0∑i∈NPi , U0≥τ 0 otherwise(9)
where the coverage threshold of the provided mission requirement is denoted by Furthermore, the coverage utility per unit of power is reflected in the energy efficiency G0. In particular, a greater G0 indicates that less transmission power is required, and hence a larger coverage utility can be achieved. As a result, the goal of this model is to discover the best UAV state. Sopt to maximize G0 [11], given by Eq. (10).
P: Sopt=arg maxG0(10)
2.2 Performance of Organized Multi -UAV Network
Given the various discrete variables in the suggested system model, an efficient solution is necessary to handle the issues of excessive computing complexity induced by large scheme cosmoses. Stimulated by advancements in wireless technologies, the model created centralized-distributed optimization architecture of UAVs networks and translated them into a cognitive decision network, where the suggested problem is handled by an optimized solution. The above works are used as a guide to solving the given model in this article [17,18].
To begin with, the mission area’s environmental factors as well as all drone information, such as positions, the ground center receives carrier transmission powers and coalition preferences, where the UAV system is charted to the virtual decision network [19].
The problem is then solved using an optimization methodology. Finally, Sopt will broadcast the obtained decision outcomes to drones, which will change their capacity for carrier transfer and fly to the prescribed sites according to Sopt. Fig. 2’s blue block diagram depicts the preceding phases. All UAVs transmit data to the ground center, which subsequently calculates decision outcomes and distributes them to the drones. Sopt is given to all UAVs, and the outcomes of the decision are carried out.
Figure 2: A schematic depicting multi-UAV coverage in a combat zone
Determine whether the UAV network’s existing coverage utility satisfies the communication needs to maximize the Coverage maximization by examining UAVs’ ideal deployment locations with their supplied maximum carrier transmission powers. If you aren’t satisfied, P doesn’t have a solution; if you are, find the best power allocation.
Coverage Probability and Cognitive Detection
The difficulty of spectrum sensing can be compared to that of binary hypothesis testing. H0 refers to the inactive user, who is the principal user, whereas H1 settles the active position. As a result, hypotheses H0 and H1 can be used to determine activeness and inactivity.
The false alarm probability (Pf = P (H1|H0)), miss detection (Pm = P (H0|H1)), and detection (Pd = P H1|H1) can all be used to assess the effectiveness of detecting the spectrum. The hypothesis is chosen based on the threshold calculated using the equation.
Conclusion 1: mentioning H0 when H0 is true (H0|H0);
Conclusion 2: mentioning H1 when H1 is true (H1|H1);
Conclusion 3: mentioning H0 when H1 is true (H0|H1);
Conclusion 4: mentioning H1 when H0 is true (H1|H0).
When the amount of energy measured exceeds the set limit, H1 is evaluated, indicating that the principal user is active/present. When the energy measured is less than the threshold value, H0 is used to indicate that the principal user is inactive or absent (Conclusion 1). Pd, Pf, and Pm’s refer to the decision on the first user’s or primary user’s activeness. When SU discovered the valid instance, i.e., Conclusion 2, Pd is the probability. Even if the PU is operational in Conclusion 3, it is still shown as inactive. This illustrates the degree to which the second user’s access chance is being squandered. The probability of miss detection (Pm = 1 − P d) is the amount of interference caused by the second user in the primary user [20–22].
Let S[n] be the received signal sample from the energy detector, which yields D, which is necessary for decisions [19], refer to Eqs. (11)–(13).
D=1Y∑Yn=1(S[n])2(11)
where Y is the no. of samples,
Pdet=P (D>T| H1),Pfalse=P (D>T|H0)(12)
Pdet=Q (T−μ1σ21),Pfalse=Q (T−μ0σ20)(13)
where T=σ20Q−1(Pfalse)+μ0
µ0,µ1,σ20,σ21 respectively the mean and divergence under H0 and H1.
The following are the sample requirements for the Pdet and Pfalse targets, shown in Eqs. (14) and (15).
Y=1SNR2(Q−1 (Pfalse)− Q−1(Pdet)√2SNR+1)2(14)
Q stands for the comprehensive Marcum Q-function.
SNR stands for signal-to-noise ratio.
Xs=tY now, where t denotes the sample time.
Xs=tSNR2(Q−1(Pfalse)−Q−1(Pdet)√2SNR+1)2(15)
The likelihood that the Primary user is inactive is P (H0), and P (H1)=1−P. (H0)
The objective function that needs to be optimized is as follows, given by Eq. (16).
{maxP(H1)TP1(Xs)+P(HO)TP0(Xs)s.t. Pdet≥ P′det(16)
The channel state is assumed to be non-line-of-sight (NLOS), (UT) is User Terminal, (BS) is Base Station, hBS and hUT are the antenna heights at the BS and UT, ‘W’ is the street width, ‘d’ is the distance between the BS and the UT, and fc is the carrier frequency in MHz (MHz). Tab. 2 contains a list of these schemes.
To get maximum radial distance coverage, as per ITU-R M.2135 standard, given in Tab. 1, and meet the threshold requirement, the ideal altitude for the primary UAV to have the minimum transmit power is shown in Fig. 4. Indeed, the ideal height is the lowest probable elevation that provides the lowermost path loss and transmits power between both the UAV and the user on the ground. In the absence of interruption, this results in preeminent excellence in communication. The best altitude and the least transmit power are also dependent on the transmission location, as shown in Fig. 4. In Rural suburban and urban environments, this finding is critical for minimizing power consumption in multi-UAV network planning.
Figure 3: Probability of coverage for a terrestrial mission cell from a specific UAV when the transmission power is taken into account
Figure 4: For urban, Suburban, and rural environments, UAV altitude vs. transmit power
In a stationary UAV deployment, transmission power can have a significant impact on coverage probability for a given UAV, as shown in Fig. 3. We’ll use a ground mission cell to capture two UAVs in separate locations to compare.
Fig. 3 shows different curves. It demonstrates that the more transmission power a UAV carries, the greater the chance of successful ground cell coverage.
As transmission power is increased, the coverage probability approaches the peak and then gradually flattens off. It’s worth noting that, additionally, there is a fixed UAV deployment, a drone’s power choices influence the possibility of its noticeable area cells being covered to capture optimal transmission power, the article design UAV n’s individual utility function.
The best position for the primary UAV to have the minimum transmission rate to give the greatest radial distance coverage while meeting the threshold requirement (11) is shown in Fig. 4. The ideal height is the lowest possible altitude that provides the lowest path loss and transmits power between the UAV and the ground user. In the absence of interruption, this leads to good information exchange. The optimal altitude and lowest transmit power are also dependent on the propagation environment, as shown in Fig. 4. The ideal altitudes for urban suburban and rural environments, for example, are 360 meters, 300 meters, and 120 meters, respectively. This finding is critical for minimizing power consumption in multi-UAV network planning.
The following is the conclusion drawn from Figs. 5 and 6. When all of the deployment scenarios in the ITU-R M.2135 are compared, the urban macro comes out ahead. The graphs show that in the high-speed deployment scenario, i.e., urban macro, the total power per subcarrier receives less FER/BER than the other two sectors [23–27].
Figure 5: For urban, suburban, and rural environments, FER vs. SNR
Figure 6: For urban, suburban, and rural environments, FER vs. SNR
In this paper, the model for multi-UAV network deployment is both energy-efficient and cost-effective. Multi-UAV coverage is a new idea that reflects UAV teamwork and improves coverage reliability and efficiency. We devised a scenario for UAV coverage to address the issue of energy scarcity by incorporating energy efficiency according to ITU-R M.2135 standard. The ideal separation distance between UAVs to avoid co-channel interference and enhance overall coverage performance has been investigated in rural, suburban, and urban settings. For this, a synchronized multi-network was created, allowing us to provide important insights into UAV communications integration. The quantity of UAVs, the weather, the deployment coordinates, or the construction of a network and spacing between UAVs all affect the coverage area performance, according to the findings of this paper. In reality, balancing the co-channel interference with a good modification of the UAV spacing can prevent coverage loss outside the intended area. This research could be expanded to UAVs operating in a massive MIMO environment. In this situation, the ideal UAV heights and separation distances for coverage by many UAVs can be identified via a multi-dimensional search. By applying the GMLSTTC to the Massive MIMO situation, the system’s performance can be significantly improved.
Acknowledgement: Ahmed Alhussen would like to thank the Deanship of Scientific Research at Majmaah University for supporting this work under Project No. R-2022-193.
Funding Statement: Ahmed Alhussen would like to thank the Deanship of Scientific Research at Majmaah University for supporting this work under Project No. R-2022-193.
Conflicts of Interest: The authors declare that they have no conflicts of interest to report regarding the present study.
References
1. B. Alidaee, H. Wang and F. Landram, “On the flexible demand assignment problems: Case of unmanned aerial vehicles,” IEEE Transactions on Automation Science & Engineering, vol. 8, no. 4, pp. 865–868, 2011. [Google Scholar]
2. A. Al-Hourani, S. Kandeepan, and S. Lardner, “Optimal LAP altitude for maximum coverage,” IEEE Wireless Communication Letters, vol. 3, no. 6, pp. 569–572, 2014. [Google Scholar]
3. J. Kosmerl and A. Vilhar, “Base stations placement optimization in wireless networks for emergency communications,” in IEEE Int. Conf. on Communications Workshops(ICC), Sydney, Australia, pp. 200–205, 2014. [Google Scholar]
4. M. Mozaffari, W. Saad, M. Bennis and M. Debbah, “Drone small cells in the clouds: Design, deployment and performance analysis,” in IEEE Global Communications Conf., vol. 46, pp. 1–6, 2015. [Google Scholar]
5. Y. Zeng, R. Zhang and T. J. Lim, “Wireless communications with unmanned aerial vehicles: Opportunities and challenges,” IEEE Communications Magazine, vol. 54, no. 5, pp. 36–42, 2016. [Google Scholar]
6. M. Mozaffari, W. Saad, M. Bennis and M. Debbah, “Efficient deployment of multiple unmanned aerial vehicles for optimal wireless coverage,” IEEE Communications Letters, vol. 20, pp. 1647–1650, 2016. [Google Scholar]
7. S. Hayat, E. Yanmaz and R. Muzaffar, “Survey on unmanned aerial vehicle networks for civil applications: A communications viewpoint,” IEEE Press, vol. 18, no. 4, pp. 2624–2661, 2016. [Google Scholar]
8. L. Gupta, R. Jain and G. Vaszkun, “Survey of important issues in UAV communication networks,” IEEE Communications Surveys & Tutorials, vol. 18, no. 2, pp. 1123–1152, 2016. [Google Scholar]
9. A. Merwaday, A. Tuncer, A. Kumbhar and I. Guvenc, “Improved throughput coverage in natural disasters: Unmanned aerial base stations for public-safety communications,” IEEE Vehicular Technology Magazine, vol. 11, no. 4, pp. 53–60, 2016. [Google Scholar]
10. M. Mozaffari, W. Saad, M. Bennis and M. Debbah, “Mobile internet of things: Can UAVs provide an energy-efficient mobile architecture,” in IEEE GLOBECOM, Washington DC, USA, pp. 1–6, 2016. [Google Scholar]
11. R. Lang, J. Wang, J. Chen, Y. Xu, Y. Yang et al., “Energy-efficient multi-UAV coverage deployment in UAV networks: A game-theoretic framework,” China Communications, vol. 15, no. 10, pp. 194–209, 2018. [Google Scholar]
12. K. A. Aziz, Z. Gan, C. Yunfei and W. Feng. “Optimum deployment of multiple UAVs for coverage area maximization in the presence of co-channel interference,” IEEE Access, vol. 7, pp. 85203–85212, 2019. [Google Scholar]
13. M. M. Azari, F. Rosas, K. C. Chen and S. Pollin, “Optimal UAV positioning for terrestrial-aerial communication in presence of fading,” in IEEE GLOBECOM, pp. 1–7, 2016. [Google Scholar]
14. M. Alzenad, A. El-Keyi, F. Lagum and H. Yanikomeroglu, “3-D placement of an unmanned aerial vehicle base station (UAV-BS) for energy-efficient maximal coverage,” IEEE Wireless Communications Letters, vol. 6, no. 4, pp. 434–437, 2011. [Google Scholar]
15. M. Alzenad, A. El-Keyi and H. Yanikomeroglu, “3D placement of an unmanned aerial vehicle base station for maximum coverage of users with different QoS requirements,” IEEE Wireless Communications Letters, vol. 7, no. 1, pp. 38–41, 2018. [Google Scholar]
16. A. Al-Hourani, K. Sithamparanathan and A. Jamalipour, “Modeling air-to-ground path loss for low altitude platforms in urban environments,’’ in IEEE Global Communications Conf., Austin, USA, pp. 2898–2904, 2015. [Google Scholar]
17. I. U. Khan, I. M. Qureshi, M. A. Aziz, T. A. Cheema and S. B. H. Shah. “Smart IoT control-based nature-inspired energy-efficient routing protocol for flying ad hoc network (FANET),” IEEE Access, vol. 8, pp. 56371–56378, 2020. [Google Scholar]
18. M. Mazari, F. Rosas and S. Pollin, “Cellular connectivity for UAVs: Network modeling, performance analysis, and design guidelines,” IEEE Transactions Wireless Communications, vol. 18, no. 7, pp. 3366–3381, 2019. [Google Scholar]
19. A. Merwaday, A. Tuncer, A. Kumbhar and I. Guvenc, “Improved throughput coverage in natural disasters: Unmanned aerial base stations for public-safety communications,” IEEE Vehicular Technology Magazine, vol. 11, no. 4, pp. 53–60, 2016. [Google Scholar]
20. N. Nie and C. Comaniciu, “Adaptive channel allocation spectrum etiquette for cognitive radio networks,” in First IEEE Int. Symp. on New Frontiers in Dynamic Spectrum Access Networks, Baltimore, USA, pp. 269–278, 2005. [Google Scholar]
21. M. Felegyhazi, M. Cagalj and J. P. Hubaux, “Efficient MAC in cognitive radio systems: A game-theoretic approach,” IEEE Transactions on Wireless Communications, vol. 8, no. 4, pp. 1984–1995, 2009. [Google Scholar]
22. Y. Xu, J. Wang, Q. Wu, A. Anpalagan and Y. D. Yao, “Opportunistic spectrum access in cognitive radio networks: Global optimization using local interaction games,” IEEE Journal of Selected Topics in Signal Processing, vol. 6, no. 2, pp. 180–194, 2012. [Google Scholar]
23. S. R. Chopra, A. Gupta and R. K. Jha, “Performance analysis of grouped multilevel space-time trellis coding technique using cognitive radio in different deployment models,” Wireless Communications and Mobile Computing, vol. 10, pp. 1–20, 2019. [Google Scholar]
24. S. R. Chopra and A. Gupta, “Error analysis of grouped multilevel space-time trellis coding with the combined application of massive MIMO and cognitive radio,” Wireless Personal Communications, vol. 117, no. 2, pp. 461–482, 2021. [Google Scholar]
25. A. Gupta and R. K. Jha, “Power optimization using optimal small cell arrangements in different deployment scenarios,” International Journal of Communication Systems, vol. 30, no. 13, pp. 1–9, 2017. [Google Scholar]
26. S. R. Chopra and A. Gupta, “Multilevel coding scheme with trellis codes as component code in 5G scenario,” in Intelligent Circuits and Systems, 1st ed., vol. 1. London, UK: CRC Press, pp. 237–243, 2021. [Google Scholar]
27. A. Gupta and R. K. Jha, “Power optimization with low complexity using scaled beamforming approach for a massive MIMO and small cell scenario,” Wireless Networks, vol. 26, no. 2, pp. 1165–1176, 2020. [Google Scholar]
Cite This Article
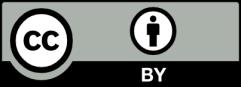
This work is licensed under a Creative Commons Attribution 4.0 International License , which permits unrestricted use, distribution, and reproduction in any medium, provided the original work is properly cited.