Open Access
ARTICLE
Bayesian Deep Learning Enabled Sentiment Analysis on Web Intelligence Applications
Department of Information Technology, College of Computer and Information Sciences, Princess Nourah bint Abdulrahman University, Riyadh, 11671, Saudi Arabia
* Corresponding Author: Abeer D. Algarni. Email:
Computers, Materials & Continua 2023, 75(2), 3399-3412. https://doi.org/10.32604/cmc.2023.026687
Received 01 January 2022; Accepted 23 February 2022; Issue published 31 March 2023
Abstract
In recent times, web intelligence (WI) has become a hot research topic, which utilizes Artificial Intelligence (AI) and advanced information technologies on the Web and Internet. The users post reviews on social media and are employed for sentiment analysis (SA), which acts as feedback to business people and government. Proper SA on the reviews helps to enhance the quality of the services and products, however, web intelligence techniques are needed to raise the company profit and user fulfillment. With this motivation, this article introduces a new modified pigeon inspired optimization based feature selection (MPIO-FS) with Bayesian deep learning (BDL), named MPIO-BDL model for SA on WI applications. The presented MPIO-BDL model initially involved preprocessing and feature extraction take place using Term Frequency—Inverse Document Frequency (TF-IDF) technique to derive a useful set of information from the user reviews. Besides, the MPIO-FS model is applied for the selection of optimal feature subsets, which helps to enhance classification accuracy and reduce computation complexity. Moreover, the BDL model is employed to allocate the proper class labels of the applied user review data. A comprehensive experimental results analysis highlighted the improved classification efficiency of the presented model.Keywords
Cite This Article
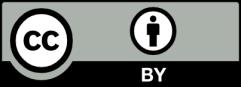