Open Access
ARTICLE
Video Frame Prediction by Joint Optimization of Direct Frame Synthesis and Optical-Flow Estimation
1 Iot and Big Data Research Center, Incheon National University, Yeonsu-gu, Incheon, 22012, Korea
2 Department of Electronics Engineering, Incheon National University, Yeonsu-gu, Incheon, 22012, Korea
* Corresponding Author: Hoon Kim. Email:
Computers, Materials & Continua 2023, 75(2), 2615-2639. https://doi.org/10.32604/cmc.2023.026086
Received 16 December 2021; Accepted 02 March 2022; Issue published 31 March 2023
Abstract
Video prediction is the problem of generating future frames by exploiting the spatiotemporal correlation from the past frame sequence. It is one of the crucial issues in computer vision and has many real-world applications, mainly focused on predicting future scenarios to avoid undesirable outcomes. However, modeling future image content and object is challenging due to the dynamic evolution and complexity of the scene, such as occlusions, camera movements, delay and illumination. Direct frame synthesis or optical-flow estimation are common approaches used by researchers. However, researchers mainly focused on video prediction using one of the approaches. Both methods have limitations, such as direct frame synthesis, usually face blurry prediction due to complex pixel distributions in the scene, and optical-flow estimation, usually produce artifacts due to large object displacements or obstructions in the clip. In this paper, we constructed a deep neural network Frame Prediction Network (FPNet-OF) with multiple-branch inputs (optical flow and original frame) to predict the future video frame by adaptively fusing the future object-motion with the future frame generator. The key idea is to jointly optimize direct RGB frame synthesis and dense optical flow estimation to generate a superior video prediction network. Using various real-world datasets, we experimentally verify that our proposed framework can produce high-level video frame compared to other state-of-the-art framework.Keywords
Cite This Article
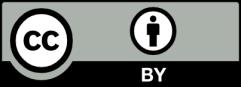