Open Access
ARTICLE
VMCTE: Visualization-Based Malware Classification Using Transfer and Ensemble Learning
1 Engineering Research Center of Digital Forensics, Ministry of Education, Nanjing University of Information Science and
Technology, Nanjing, 210044, China
2 School of Computer and Software, Nanjing University of Information Science and Technology, Nanjing, 210044, China
* Corresponding Author: Zhiguo Chen. Email:
Computers, Materials & Continua 2023, 75(2), 4445-4465. https://doi.org/10.32604/cmc.2023.038639
Received 22 December 2022; Accepted 17 February 2023; Issue published 31 March 2023
Abstract
The Corona Virus Disease 2019 (COVID-19) effect has made telecommuting and remote learning the norm. The growing number of Internet-connected devices provides cyber attackers with more attack vectors. The development of malware by criminals also incorporates a number of sophisticated obfuscation techniques, making it difficult to classify and detect malware using conventional approaches. Therefore, this paper proposes a novel visualization-based malware classification system using transfer and ensemble learning (VMCTE). VMCTE has a strong anti-interference ability. Even if malware uses obfuscation, fuzzing, encryption, and other techniques to evade detection, it can be accurately classified into its corresponding malware family. Unlike traditional dynamic and static analysis techniques, VMCTE does not require either reverse engineering or the aid of domain expert knowledge. The proposed classification system combines three strong deep convolutional neural networks (ResNet50, MobilenetV1, and MobilenetV2) as feature extractors, lessens the dimension of the extracted features using principal component analysis, and employs a support vector machine to establish the classification model. The semantic representations of malware images can be extracted using various convolutional neural network (CNN) architectures, obtaining higher-quality features than traditional methods. Integrating fine-tuned and non-fine-tuned classification models based on transfer learning can greatly enhance the capacity to classify various families of malware. The experimental findings on the Malimg dataset demonstrate that VMCTE can attain 99.64%, 99.64%, 99.66%, and 99.64% accuracy, F1-score, precision, and recall, respectively.Keywords
Cite This Article
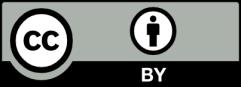
This work is licensed under a Creative Commons Attribution 4.0 International License , which permits unrestricted use, distribution, and reproduction in any medium, provided the original work is properly cited.