Open Access
ARTICLE
Classifying Misinformation of User Credibility in Social Media Using Supervised Learning
1 Department of Computer Science, Center of Excellence in Artificial Intelligence (CoE-AI), Bahria University,
Islamabad, 44000, Pakistan
2 Department of Computer Science and Information Technology, University of Engineering and Technology,
Peshawar, 25000, Pakistan
* Corresponding Authors: Muhammad Asfand-e-yar. Email: ; Qadeer Hashir. Email:
Computers, Materials & Continua 2023, 75(2), 2921-2938. https://doi.org/10.32604/cmc.2023.034741
Received 26 July 2022; Accepted 07 January 2023; Issue published 31 March 2023
Abstract
The growth of the internet and technology has had a significant effect on social interactions. False information has become an important research topic due to the massive amount of misinformed content on social networks. It is very easy for any user to spread misinformation through the media. Therefore, misinformation is a problem for professionals, organizers, and societies. Hence, it is essential to observe the credibility and validity of the News articles being shared on social media. The core challenge is to distinguish the difference between accurate and false information. Recent studies focus on News article content, such as News titles and descriptions, which has limited their achievements. However, there are two ordinarily agreed-upon features of misinformation: first, the title and text of an article, and second, the user engagement. In the case of the News context, we extracted different user engagements with articles, for example, tweets, i.e., read-only, user retweets, likes, and shares. We calculate user credibility and combine it with article content with the user’s context. After combining both features, we used three Natural language processing (NLP) feature extraction techniques, i.e., Term Frequency-Inverse Document Frequency (TF-IDF), Count-Vectorizer (CV), and Hashing-Vectorizer (HV). Then, we applied different machine learning classifiers to classify misinformation as real or fake. Therefore, we used a Support Vector Machine (SVM), Naive Byes (NB), Random Forest (RF), Decision Tree (DT), Gradient Boosting (GB), and K-Nearest Neighbors (KNN). The proposed method has been tested on a real-world dataset, i.e., “fakenewsnet”. We refine the fakenewsnet dataset repository according to our required features. The dataset contains 23000+ articles with millions of user engagements. The highest accuracy score is 93.4%. The proposed model achieves its highest accuracy using count vector features and a random forest classifier. Our discoveries confirmed that the proposed classifier would effectively classify misinformation in social networks.Keywords
Cite This Article
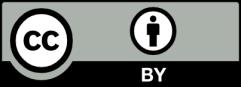
This work is licensed under a Creative Commons Attribution 4.0 International License , which permits unrestricted use, distribution, and reproduction in any medium, provided the original work is properly cited.