Open Access
ARTICLE
MCMOD: The Multi-Category Large-Scale Dataset for Maritime Object Detection
1 Institute of Remote Sensing and Geographic Information System, Peking University, Beijing, 100871, China
2 Department of Electronic Engineering, Tsinghua University, Beijing, 100084, China
3 Institute of Acoustics, Chinese Academy of Sciences, Beijing, 100190, China
* Corresponding Author: Zihao Sun. Email:
Computers, Materials & Continua 2023, 75(1), 1657-1669. https://doi.org/10.32604/cmc.2023.036558
Received 04 October 2022; Accepted 14 December 2022; Issue published 06 February 2023
Abstract
The marine environment is becoming increasingly complex due to the various marine vehicles, and the diversity of maritime objects poses a challenge to marine environmental governance. Maritime object detection technology plays an important role in this segment. In the field of computer vision, there is no sufficiently comprehensive public dataset for maritime objects in the contrast to the automotive application domain. The existing maritime datasets either have no bounding boxes (which are made for object classification) or cover limited varieties of maritime objects. To fulfil the vacancy, this paper proposed the Multi-Category Large-Scale Dataset for Maritime Object Detection (MCMOD) which is collected by 3 onshore video cameras that capture data under various environmental conditions such as fog, rain, evening, etc. The whole dataset consists of 16,166 labelled images along with 98,590 maritime objects which are classified into 10 classes. Compared with the existing maritime datasets, MCMOD contains a relatively balanced quantity of objects of different sizes (in the view). To evaluate MCMOD, this paper applied several state-of-the-art object detection approaches from computer vision research on it and compared their performances. Moreover, a comparison between MCMOD and an existing maritime dataset was conducted. Experimental results indicate that the proposed dataset classifies more types of maritime objects and covers more small-scale objects, which can facilitate the trained detectors to recognize more types of maritime objects and detect maritime objects over a relatively long distance. The obtained results also show that the adopted approaches need to be further improved to enhance their capabilities in the maritime domain.Keywords
Cite This Article
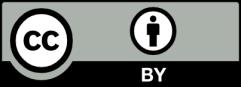