Open Access
ARTICLE
Machine Learning Techniques for Detecting Phishing URL Attacks
1 Department of Cyber Security, College of Engineering and Information Technology, Buraydah Private Colleges, Buraydah, 51418, Kingdom of Saudi Arabia
2 Faculty of Computers and Information, Kafrelsheikh University, Kafrelsheikh, 33516, Egypt
3 Faculty of Artificial Intelligence, Kafrelsheikh University, Kafrelsheikh, 33516, Egypt
4 Delta Higher Institute of Engineering and Technology, Mansoura, 35111, Egypt
5 Faculty of Computers and Information, Fayoum University, Fayoum, Egypt
* Corresponding Author: Mahmoud Y. Shams. Email:
Computers, Materials & Continua 2023, 75(1), 1271-1290. https://doi.org/10.32604/cmc.2023.036422
Received 29 September 2022; Accepted 09 December 2022; Issue published 06 February 2023
Abstract
Cyber Attacks are critical and destructive to all industry sectors. They affect social engineering by allowing unapproved access to a Personal Computer (PC) that breaks the corrupted system and threatens humans. The defense of security requires understanding the nature of Cyber Attacks, so prevention becomes easy and accurate by acquiring sufficient knowledge about various features of Cyber Attacks. Cyber-Security proposes appropriate actions that can handle and block attacks. A phishing attack is one of the cybercrimes in which users follow a link to illegal websites that will persuade them to divulge their private information. One of the online security challenges is the enormous number of daily transactions done via phishing sites. As Cyber-Security have a priority for all organizations, Cyber-Security risks are considered part of an organization’s risk management process. This paper presents a survey of different modern machine-learning approaches that handle phishing problems and detect with high-quality accuracy different phishing attacks. A dataset consisting of more than 11000 websites from the Kaggle dataset was utilized and studying the effect of 30 website features and the resulting class label indicating whether or not it is a phishing website (1 or −1). Furthermore, we determined the confusion matrices of Machine Learning models: Neural Networks (NN), Naïve Bayes, and Adaboost, and the results indicated that the accuracies achieved were 90.23%, 92.97%, and 95.43%, respectively.Keywords
Cite This Article
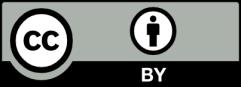