Open Access
ARTICLE
A Semantic Adversarial Network for Detection and Classification of Myopic Maculopathy
1 College of Computer and Information Sciences, Imam Mohammad Ibn Saud Islamic University (IMSIU), Riyadh, 11432, Saudi Arabia
2 Department of Computer Science, Quaid-i-Azam University, Islamabad, 44000, Pakistan
* Corresponding Author: Abdul Rauf Baig. Email:
Computers, Materials & Continua 2023, 75(1), 1483-1499. https://doi.org/10.32604/cmc.2023.036366
Received 28 September 2022; Accepted 04 November 2022; Issue published 06 February 2023
Abstract
The diagnosis of eye disease through deep learning (DL) technology is the latest trend in the field of artificial intelligence (AI). Especially in diagnosing pathologic myopia (PM) lesions, the implementation of DL is a difficult task because of the classification complexity and definition system of PM. However, it is possible to design an AI-based technique that can identify PM automatically and help doctors make relevant decisions. To achieve this objective, it is important to have adequate resources such as a high-quality PM image dataset and an expert team. The primary aim of this research is to design and train the DLs to automatically identify and classify PM into different classes. In this article, we have developed a new class of DL models (SAN-FSL) for the segmentation and detection of PM through semantic adversarial networks (SAN) and few-short learning (FSL) methods, respectively. Compared to DL methods, the conventional segmentation methods use supervised learning models, so they (a) require a lot of data for training and (b) fixed weights are used after the completion of the training process. To solve such problems, the FSL technique was employed for model training with few samples. The ability of FSL learning in UNet architectures is being explored, and to fine-tune the weights, a few new samples are being provided to the UNet. The outcomes show improvement in the detection area and classification of PM stages. Betterment in the result is observed by sensitivity (SE) of 95%, specificity (SP) of 96%, and area under the receiver operating curve (AUC) of 98%, and the higher F1-score is achieved using 10-fold cross-validation. Furthermore, the obtained results confirmed the superiority of the SAN-FSL method.Keywords
Cite This Article
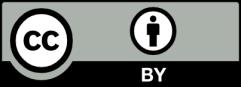
This work is licensed under a Creative Commons Attribution 4.0 International License , which permits unrestricted use, distribution, and reproduction in any medium, provided the original work is properly cited.