Open Access
ARTICLE
Dynamic Behavior-Based Churn Forecasts in the Insurance Sector
School of Computer Science and Engineering (SCOPE), VIT-AP University, Amaravati, 522237, India
* Corresponding Author: Nagendra Panini Challa. Email:
Computers, Materials & Continua 2023, 75(1), 977-997. https://doi.org/10.32604/cmc.2023.036098
Received 16 September 2022; Accepted 14 December 2022; Issue published 06 February 2023
Abstract
In the insurance sector, a massive volume of data is being generated on a daily basis due to a vast client base. Decision makers and business analysts emphasized that attaining new customers is costlier than retaining existing ones. The success of retention initiatives is determined not only by the accuracy of forecasting churners but also by the timing of the forecast. Previous works on churn forecast presented models for anticipating churn quarterly or monthly with an emphasis on customers’ static behavior. This paper’s objective is to calculate daily churn based on dynamic variations in client behavior. Training excellent models to further identify potential churning customers helps insurance companies make decisions to retain customers while also identifying areas for improvement. Thus, it is possible to identify and analyse clients who are likely to churn, allowing for a reduction in the cost of support and maintenance. Binary Golden Eagle Optimizer (BGEO) is used to select optimal features from the datasets in a preprocessing step. As a result, this research characterized the customer's daily behavior using various models such as RFM (Recency, Frequency, Monetary), Multivariate Time Series (MTS), Statistics-based Model (SM), Survival analysis (SA), Deep learning (DL) based methodologies such as Recurrent Neural Network (RNN), Long Short-Term Memory (LSTM), Gated Recurrent Unit (GRU), and Customized Extreme Learning Machine (CELM) are framed the problem of daily forecasting using this description. It can be concluded that all models produced better overall outcomes with only slight variations in performance measures. The proposed CELM outperforms all other models in terms of accuracy (96.4).Keywords
Cite This Article
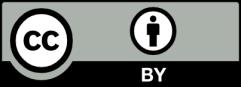