Open Access
ARTICLE
Machine Learning Based Classifiers for QoE Prediction Framework in Video Streaming over 5G Wireless Networks
School of Electronics Engineering, Vellore Institute of Technology, Chennai, 600127, India
* Corresponding Author: P. Vetrivelan. Email:
Computers, Materials & Continua 2023, 75(1), 1919-1939. https://doi.org/10.32604/cmc.2023.036013
Received 13 September 2022; Accepted 04 November 2022; Issue published 06 February 2023
Abstract
Recently, the combination of video services and 5G networks have been gaining attention in the wireless communication realm. With the brisk advancement in 5G network usage and the massive popularity of three-dimensional video streaming, the quality of experience (QoE) of video in 5G systems has been receiving overwhelming significance from both customers and service provider ends. Therefore, effectively categorizing QoE-aware video streaming is imperative for achieving greater client satisfaction. This work makes the following contribution: First, a simulation platform based on NS-3 is introduced to analyze and improve the performance of video services. The simulation is formulated to offer real-time measurements, saving the expensive expenses associated with real-world equipment. Second, A valuable framework for QoE-aware video streaming categorization is introduced in 5G networks based on machine learning (ML) by incorporating the hyperparameter tuning (HPT) principle. It implements an enhanced hyperparameter tuning (EHPT) ensemble and decision tree (DT) classifier for video streaming categorization. The performance of the ML approach is assessed by considering precision, accuracy, recall, and computation time metrics for manifesting the superiority of these classifiers regarding video streaming categorization. This paper demonstrates that our ML classifiers achieve QoE prediction accuracy of 92.59% for (EHPT) ensemble and 87.037% for decision tree (DT) classifiers.Keywords
Cite This Article
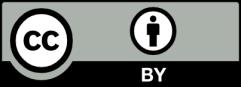
This work is licensed under a Creative Commons Attribution 4.0 International License , which permits unrestricted use, distribution, and reproduction in any medium, provided the original work is properly cited.