Open Access
ARTICLE
A Deep Learning-Based Crowd Counting Method and System Implementation on Neural Processing Unit Platform
Nanjing University of Posts and Telecommunications, Nanjing, P210023, China
* Corresponding Author: Meng Wu. Email:
Computers, Materials & Continua 2023, 75(1), 493-512. https://doi.org/10.32604/cmc.2023.035974
Received 12 September 2022; Accepted 12 November 2022; Issue published 06 February 2023
Abstract
In this paper, a deep learning-based method is proposed for crowd-counting problems. Specifically, by utilizing the convolution kernel density map, the ground truth is generated dynamically to enhance the feature-extracting ability of the generator model. Meanwhile, the “cross stage partial” module is integrated into congested scene recognition network (CSRNet) to obtain a lightweight network model. In addition, to compensate for the accuracy drop owing to the lightweight model, we take advantage of “structured knowledge transfer” to train the model in an end-to-end manner. It aims to accelerate the fitting speed and enhance the learning ability of the student model. The crowd-counting system solution for edge computing is also proposed and implemented on an embedded device equipped with a neural processing unit. Simulations demonstrate the performance improvement of the proposed solution in terms of model size, processing speed and accuracy. The performance on the Venice dataset shows that the mean absolute error (MAE) and the root mean squared error (RMSE) of our model drop by 32.63% and 39.18% compared with CSRNet. Meanwhile, the performance on the ShanghaiTech PartB dataset reveals that the MAE and the RMSE of our model are close to those of CSRNet. Therefore, we provide a novel embedded platform system scheme for public safety pre-warning applications.Keywords
Cite This Article
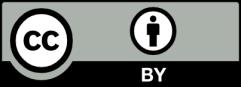
This work is licensed under a Creative Commons Attribution 4.0 International License , which permits unrestricted use, distribution, and reproduction in any medium, provided the original work is properly cited.