Open Access
ARTICLE
Human Verification over Activity Analysis via Deep Data Mining
1 Senior Member, IEEE, Seattle, 98103, USA
2 Department of Software Engineering, Capital University of Science and Technology (CUST), Islamabad, 44000, Pakistan
* Corresponding Author: Kumar Abhishek. Email:
Computers, Materials & Continua 2023, 75(1), 1391-1409. https://doi.org/10.32604/cmc.2023.035894
Received 08 September 2022; Accepted 08 December 2022; Issue published 06 February 2023
Abstract
Human verification and activity analysis (HVAA) are primarily employed to observe, track, and monitor human motion patterns using red-green-blue (RGB) images and videos. Interpreting human interaction using RGB images is one of the most complex machine learning tasks in recent times. Numerous models rely on various parameters, such as the detection rate, position, and direction of human body components in RGB images. This paper presents robust human activity analysis for event recognition via the extraction of contextual intelligence-based features. To use human interaction image sequences as input data, we first perform a few denoising steps. Then, human-to-human analyses are employed to deliver more precise results. This phase follows feature engineering techniques, including diverse feature selection. Next, we used the graph mining method for feature optimization and AdaBoost for classification. We tested our proposed HVAA model on two benchmark datasets. The testing of the proposed HVAA system exhibited a mean accuracy of 92.15% for the Sport Videos in the Wild (SVW) dataset. The second benchmark dataset, UT-interaction, had a mean accuracy of 92.83%. Therefore, these results demonstrated a better recognition rate and outperformed other novel techniques in body part tracking and event detection. The proposed HVAA system can be utilized in numerous real-world applications including, healthcare, surveillance, task monitoring, atomic actions, gesture and posture analysis.Keywords
Cite This Article
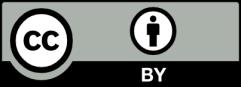
This work is licensed under a Creative Commons Attribution 4.0 International License , which permits unrestricted use, distribution, and reproduction in any medium, provided the original work is properly cited.