Open Access
ARTICLE
Attenuate Class Imbalance Problem for Pneumonia Diagnosis Using Ensemble Parallel Stacked Pre-Trained Models
School of Computer Science and Engineering, Vellore Institute of Technology, Chennai, 600127, India
* Corresponding Author: Harini Sriraman. Email:
Computers, Materials & Continua 2023, 75(1), 891-909. https://doi.org/10.32604/cmc.2023.035848
Received 06 September 2022; Accepted 19 November 2022; Issue published 06 February 2023
Abstract
Pneumonia is an acute lung infection that has caused many fatalities globally. Radiologists often employ chest X-rays to identify pneumonia since they are presently the most effective imaging method for this purpose. Computer-aided diagnosis of pneumonia using deep learning techniques is widely used due to its effectiveness and performance. In the proposed method, the Synthetic Minority Oversampling Technique (SMOTE) approach is used to eliminate the class imbalance in the X-ray dataset. To compensate for the paucity of accessible data, pre-trained transfer learning is used, and an ensemble Convolutional Neural Network (CNN) model is developed. The ensemble model consists of all possible combinations of the MobileNetv2, Visual Geometry Group (VGG16), and DenseNet169 models. MobileNetV2 and DenseNet169 performed well in the Single classifier model, with an accuracy of 94%, while the ensemble model (MobileNetV2+DenseNet169) achieved an accuracy of 96.9%. Using the data synchronous parallel model in Distributed Tensorflow, the training process accelerated performance by 98.6% and outperformed other conventional approaches.Keywords
Cite This Article
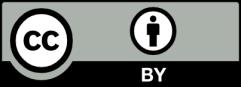
This work is licensed under a Creative Commons Attribution 4.0 International License , which permits unrestricted use, distribution, and reproduction in any medium, provided the original work is properly cited.