Open Access
ARTICLE
A Levenberg–Marquardt Based Neural Network for Short-Term Load Forecasting
1 School of Computer Science Guangzhou University, Guangzhou, 510006, Guangdong Province, China
2 Department of Computer Science, University of Agriculture, Faisalabad, 38000, Pakistan
3 Department of Computer Science, Government College Women University, Faisalabad, 38000, Pakistan
* Corresponding Author: Guojun Wang. Email:
Computers, Materials & Continua 2023, 75(1), 1783-1800. https://doi.org/10.32604/cmc.2023.035736
Received 01 September 2022; Accepted 14 December 2022; Issue published 06 February 2023
Abstract
Short-term load forecasting (STLF) is part and parcel of the efficient working of power grid stations. Accurate forecasts help to detect the fault and enhance grid reliability for organizing sufficient energy transactions. STLF ranges from an hour ahead prediction to a day ahead prediction. Various electric load forecasting methods have been used in literature for electricity generation planning to meet future load demand. A perfect balance regarding generation and utilization is still lacking to avoid extra generation and misusage of electric load. Therefore, this paper utilizes Levenberg–Marquardt (LM) based Artificial Neural Network (ANN) technique to forecast the short-term electricity load for smart grids in a much better, more precise, and more accurate manner. For proper load forecasting, we take the most critical weather parameters along with historical load data in the form of time series grouped into seasons, i.e., winter and summer. Further, the presented model deals with each season’s load data by splitting it into weekdays and weekends. The historical load data of three years have been used to forecast week-ahead and day-ahead load demand after every thirty minutes making load forecast for a very short period. The proposed model is optimized using the Levenberg-Marquardt backpropagation algorithm to achieve results with comparable statistics. Mean Absolute Percent Error (MAPE), Root Mean Squared Error (RMSE), R2, and R are used to evaluate the model. Compared with other recent machine learning-based mechanisms, our model presents the best experimental results with MAPE and R2 scores of 1.3 and 0.99, respectively. The results prove that the proposed LM-based ANN model performs much better in accuracy and has the lowest error rates as compared to existing work.Keywords
Cite This Article
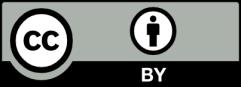
This work is licensed under a Creative Commons Attribution 4.0 International License , which permits unrestricted use, distribution, and reproduction in any medium, provided the original work is properly cited.