Open Access
ARTICLE
Federated Feature Concatenate Method for Heterogeneous Computing in Federated Learning
1 Department of Information and Computer Engineering, Chung Yuan Christian University, Taoyuan, Taiwan
2 Department of Computer Science and Information Engineering, Asia University, Taichung, Taiwan
3 Department of Medical Research, China Medical University Hospital, China Medical University, Taichung, Taiwan
4 Department of Computer Science & Communication Engineering, Providence University, Taichung, Taiwan
5 Institute of Biomedical Informatics, National Yang Ming Chiao Tung University, Taipei, Taiwan
* Corresponding Author: Che-Lun Hung. Email:
Computers, Materials & Continua 2023, 75(1), 351-371. https://doi.org/10.32604/cmc.2023.035720
Received 01 September 2022; Accepted 17 November 2022; Issue published 06 February 2023
Abstract
Federated learning is an emerging machine learning technique that enables clients to collaboratively train a deep learning model without uploading raw data to the aggregation server. Each client may be equipped with different computing resources for model training. The client equipped with a lower computing capability requires more time for model training, resulting in a prolonged training time in federated learning. Moreover, it may fail to train the entire model because of the out-of-memory issue. This study aims to tackle these problems and propose the federated feature concatenate (FedFC) method for federated learning considering heterogeneous clients. FedFC leverages the model splitting and feature concatenate for offloading a portion of the training loads from clients to the aggregation server. Each client in FedFC can collaboratively train a model with different cutting layers. Therefore, the specific features learned in the deeper layer of the server-side model are more identical for the data class classification. Accordingly, FedFC can reduce the computation loading for the resource-constrained client and accelerate the convergence time. The performance effectiveness is verified by considering different dataset scenarios, such as data and class imbalance for the participant clients in the experiments. The performance impacts of different cutting layers are evaluated during the model training. The experimental results show that the co-adapted features have a critical impact on the adequate classification of the deep learning model. Overall, FedFC not only shortens the convergence time, but also improves the best accuracy by up to 5.9% and 14.5% when compared to conventional federated learning and splitfed, respectively. In conclusion, the proposed approach is feasible and effective for heterogeneous clients in federated learning.Keywords
Cite This Article
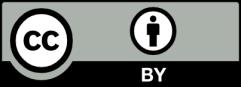