Open Access
ARTICLE
Multimodal Fused Deep Learning Networks for Domain Specific Image Similarity Search
Research and Development, AItheNutrigene, Seoul, 06132, Korea
* Corresponding Author: Donghun Lee. Email:
Computers, Materials & Continua 2023, 75(1), 243-258. https://doi.org/10.32604/cmc.2023.035716
Received 01 September 2022; Accepted 09 November 2022; Issue published 06 February 2023
Abstract
The exponential increase in data over the past few years, particularly in images, has led to more complex content since visual representation became the new norm. E-commerce and similar platforms maintain large image catalogues of their products. In image databases, searching and retrieving similar images is still a challenge, even though several image retrieval techniques have been proposed over the decade. Most of these techniques work well when querying general image databases. However, they often fail in domain-specific image databases, especially for datasets with low intraclass variance. This paper proposes a domain-specific image similarity search engine based on a fused deep learning network. The network is comprised of an improved object localization module, a classification module to narrow down search options and finally a feature extraction and similarity calculation module. The network features both an offline stage for indexing the dataset and an online stage for querying. The dataset used to evaluate the performance of the proposed network is a custom domain-specific dataset related to cosmetics packaging gathered from various online platforms. The proposed method addresses the intraclass variance problem with more precise object localization and the introduction of top result reranking based on object contours. Finally, quantitative and qualitative experiment results are presented, showing improved image similarity search performance.Keywords
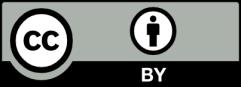