Open Access
ARTICLE
An Automated System for Early Prediction of Miscarriage in the First Trimester Using Machine Learning
1 Department of Computer Science, College of Computer Science and Information Technology, Imam Abdulrahman Bin Faisal University, P.O. Box 1982, Dammam, 31441, Saudi Arabia
2 Computer Science Department, College of Computer and Information Systems, Umm Al-Qura University, Makkah, 21955, Saudi Arabia
3 Department of Computer Engineering, College of Computer Science and Information Technology, Imam Abdulrahman Bin Faisal University, P.O. Box 1982, Dammam, 31441, Saudi Arabia
4 Department of Obstetrics and Gynecology, College of Medicine, Imam Abdulrahman Bin Faisal University, Dammam, Saudi Arabia
* Corresponding Author: Dorieh M. Alomari. Email:
Computers, Materials & Continua 2023, 75(1), 1291-1304. https://doi.org/10.32604/cmc.2023.035710
Received 01 September 2022; Accepted 08 December 2022; Issue published 06 February 2023
Abstract
Currently, the risk factors of pregnancy loss are increasing and are considered a major challenge because they vary between cases. The early prediction of miscarriage can help pregnant ladies to take the needed care and avoid any danger. Therefore, an intelligent automated solution must be developed to predict the risk factors for pregnancy loss at an early stage to assist with accurate and effective diagnosis. Machine learning (ML)-based decision support systems are increasingly used in the healthcare sector and have achieved notable performance and objectiveness in disease prediction and prognosis. Thus, we developed a model to help obstetricians predict the probability of miscarriage using ML. And support their decisions and expectations about pregnancy status by providing an easy, automated way to predict miscarriage at early stages using ML tools and techniques. Although many published papers proposed similar models, none of them used Saudi clinical data. Our proposed solution used ML classification algorithms to build a miscarriage prediction model. Four classifiers were used in this study: decision tree (DT), random forest (RF), k-nearest neighbor (KNN), and gradient boosting (GB). Accuracy, Precision, Recall, F1-score, and receiver operating characteristic area under the curve (ROC-AUC) were used to evaluate the proposed model. The results showed that GB overperformed the other classifiers with an accuracy of 93.4% and ROC-AUC of 97%. This proposed model can assist in the early identification of at-risk pregnant women to avoid miscarriage in the first trimester and will improve the healthcare sector in Saudi Arabia.Keywords
Cite This Article
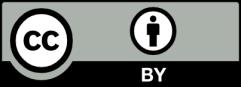
This work is licensed under a Creative Commons Attribution 4.0 International License , which permits unrestricted use, distribution, and reproduction in any medium, provided the original work is properly cited.