Open Access
ARTICLE
Faster Metallic Surface Defect Detection Using Deep Learning with Channel Shuffling
1 Department of Robot System Engineering, Tongmyong University, Busan, 48520, Korea
2 School of Artificial Intelligence, Tongmyong University, Busan, 48520, Korea
* Corresponding Author: Hyunsik Ahn. Email:
Computers, Materials & Continua 2023, 75(1), 1847-1861. https://doi.org/10.32604/cmc.2023.035698
Received 31 August 2022; Accepted 24 October 2022; Issue published 06 February 2023
Abstract
Deep learning has been constantly improving in recent years, and a significant number of researchers have devoted themselves to the research of defect detection algorithms. Detection and recognition of small and complex targets is still a problem that needs to be solved. The authors of this research would like to present an improved defect detection model for detecting small and complex defect targets in steel surfaces. During steel strip production, mechanical forces and environmental factors cause surface defects of the steel strip. Therefore, the detection of such defects is key to the production of high-quality products. Moreover, surface defects of the steel strip cause great economic losses to the high-tech industry. So far, few studies have explored methods of identifying the defects, and most of the currently available algorithms are not sufficiently effective. Therefore, this study presents an improved real-time metallic surface defect detection model based on You Only Look Once (YOLOv5) specially designed for small networks. For the smaller features of the target, the conventional part is replaced with a depth-wise convolution and channel shuffle mechanism. Then assigning weights to Feature Pyramid Networks (FPN) output features and fusing them, increases feature propagation and the network’s characterization ability. The experimental results reveal that the improved proposed model outperforms other comparable models in terms of accuracy and detection time. The precision of the proposed model achieved by mAP@0.5 is 77.5% on the Northeastern University, Dataset (NEU-DET) and 70.18% on the GC10-DET datasets.Keywords
Cite This Article
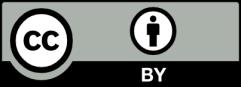
This work is licensed under a Creative Commons Attribution 4.0 International License , which permits unrestricted use, distribution, and reproduction in any medium, provided the original work is properly cited.