Open Access
ARTICLE
Enhanced Clustering Based OSN Privacy Preservation to Ensure k-Anonymity, t-Closeness, l-Diversity, and Balanced Privacy Utility
1 Department of CSE, Lovely Professional University, Phagwara, 144411, India
2 Department of CSE, Symbiosis Institute of Technology (SIT), Affiliated to Symbiosis International (Deemed University), Pune, 412115, India
3 School of Computer Applications, Lovely Professional University, Phagwara, 144411, India
4 Learning & Development, Persistent University, Persistent Systems, Pune, 411057, India
* Corresponding Author: Rupali Gangarde. Email:
Computers, Materials & Continua 2023, 75(1), 2171-2190. https://doi.org/10.32604/cmc.2023.035559
Received 25 August 2022; Accepted 08 December 2022; Issue published 06 February 2023
Abstract
Online Social Networks (OSN) sites allow end-users to share a great deal of information, which may also contain sensitive information, that may be subject to commercial or non-commercial privacy attacks. As a result, guaranteeing various levels of privacy is critical while publishing data by OSNs. The clustering-based solutions proved an effective mechanism to achieve the privacy notions in OSNs. But fixed clustering limits the performance and scalability. Data utility degrades with increased privacy, so balancing the privacy utility trade-off is an open research issue. The research has proposed a novel privacy preservation model using the enhanced clustering mechanism to overcome this issue. The proposed model includes phases like pre-processing, enhanced clustering, and ensuring privacy preservation. The enhanced clustering algorithm is the second phase where authors modified the existing fixed k-means clustering using the threshold approach. The threshold value is determined based on the supplied OSN data of edges, nodes, and user attributes. Clusters are k-anonymized with multiple graph properties by a novel one-pass algorithm. After achieving the k-anonymity of clusters, optimization was performed to achieve all privacy models, such as k-anonymity, t-closeness, and l-diversity. The proposed privacy framework achieves privacy of all three network components, i.e., link, node, and user attributes, with improved utility. The authors compare the proposed technique to underlying methods using OSN Yelp and Facebook datasets. The proposed approach outperformed the underlying state of art methods for Degree of Anonymization, computational efficiency, and information loss.Keywords
Cite This Article
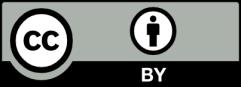
This work is licensed under a Creative Commons Attribution 4.0 International License , which permits unrestricted use, distribution, and reproduction in any medium, provided the original work is properly cited.