Open Access
ARTICLE
Construction and Application of Cloud Computing Model for Reciprocal and Collaborative Knowledge Management
1 Business School, Hohai University, Nanjing, 211100, China
2 Network and Information Technology Center, Hohai University, Nanjing, 210098, China
* Corresponding Author: Jingqi Li. Email:
Computers, Materials & Continua 2023, 75(1), 1119-1137. https://doi.org/10.32604/cmc.2023.035369
Received 18 August 2022; Accepted 08 December 2022; Issue published 06 February 2023
Abstract
Promoting the co-constructing and sharing of organizational knowledge and improving organizational performance have always been the core research subject of knowledge management. Existing research focuses on the construction of knowledge management systems and knowledge sharing and transfer mechanisms. With the rapid development and application of cloud computing and big data technology, knowledge management is faced with many problems, such as how to combine with the new generation of information technology, how to achieve integration with organizational business processes, and so on. To solve such problems, this paper proposes a reciprocal collaborative knowledge management model (RCKM model) based on cloud computing technology, reciprocity theory, and collaboration technology. RCKM model includes project group management and cloud computing technology, which can realize management, finance, communication, and quality assurance of multiple projects and solve the problem of business integration with knowledge management. This paper designs evaluation methods of tacit knowledge and reciprocity preference based on the Bayesian formula and analyzes their effect with simulation data. The methods can provide quantitative support for the integration of knowledge management and business management to realize reciprocity and collaboration in the RCKM model. The research found that RCKM model can fully use cloud computing technology to promote the integration of knowledge management and organizational business, and the evaluation method based on the Bayesian formula can provide relatively accurate data support for the evaluation and selection of project team members.Keywords
Knowledge management is a cross-research field of management and information science, which is widely used in all fields of society. The development of knowledge management has brought great changes to organizational behavior and promoted the progress and development of organizational management technology. In the new economic system, knowledge is the most meaningful one of the manufacturing resources, knowledge capital is the main source of core enterprise competence, and knowledge management is the necessary means to realize the value function of knowledge capital [1]. In application practice, knowledge management with knowledge resources as the core is driven by information technology and focuses on explicit knowledge management. Knowledge management with learning organization as the core emphasizes the generation and sharing of knowledge and focuses on tacit knowledge management [2]. Costa et al. [3] analyzed 45 papers on knowledge management process and innovation, the result showed that all knowledge processes can directly support innovation, among which knowledge creation and knowledge application are the two core processes affecting innovation, while knowledge acquisition and knowledge sharing are the most frequently studied knowledge processes. Zanker et al. [4] tried to integrate the methodology of system dynamics into the domain of knowledge management and believe that system dynamics has great potential to support the creation of new knowledge through the creation of models and simulation. Zhang et al. [5] believe that knowledge management is increasingly showing the characteristics of digitalization, and traditional knowledge management needs to transit to digital knowledge management, to identify and integrate knowledge independently and intelligently, to significantly improve organizational decision-making.
With the deepening of knowledge management research and the promotion of its application, knowledge management is more and more widely used in enterprises, but there are many problems with the current application of knowledge management. At present, the new generation of information technology with big data, cloud computing, and artificial intelligence as the core is developing rapidly. Big data technology can directly provide decision support through data processing, and artificial intelligence can support automatic decision-making. Therefore, what role knowledge management can play and how to combine with these technologies are in urgent need of theoretical support and guidance. On the other hand, most of the existing research focuses on how to effectively share and spread tacit knowledge, while there is less research on the integration of knowledge management and business processes. Compared with business processes, knowledge management does not produce direct organizational performance. Therefore, the integration with business processes can make the role of knowledge management more explicit and obtain more support. In the existing literature, there are few studies on the evaluation of tacit knowledge, and few studies on tacit knowledge sharing take the reciprocal preference of knowledge-sharing subjects into account. But in collaborative team knowledge management, how to realize the effective diffusion of tacit knowledge of team members is an important problem needed to be solved [6]. The first problem to be solved in knowledge transfer is the identification of tacit knowledge, that is, how to find and evaluate the tacit knowledge of team members. These problems need to break through the limitations of traditional theories, further introduce new theories, methods, and technologies, and combine knowledge management with actual business processes, to truly play its role and improve the performance of organizations.
In the past literature, there were fewer discussions on the integration of knowledge management and business management, and most of them started from knowledge management itself to seeking knowledge management application solutions. This paper provides a new reference framework. The reciprocal and collaborative knowledge management framework based on cloud computing realizes the integration of knowledge management and business process, quantifies the reciprocal preference and tacit knowledge of organizational members, and improves organizational performance through team collaboration.
This paper consists of six sections. The first section introduces the background, problem statement, and our contributions. Section 2 discusses the internal relationship between knowledge management, reciprocity theory, and computer-supported cooperative work theory through literature reviews of relevant work. Section 3 describes the method and motivation of this research. Section 4 designs and describes the reciprocal and collaborative knowledge management framework based on cloud computing. Section 5 analyzes and designs the evaluation methods of reciprocity preference and tacit knowledge. Finally, we draw conclusions in Section 6.
2.1 Influence of Collaboration Technology on Knowledge Management
In the modern information technology environment, collaboration technology is to use various IT hardware, software, and processes to enable organizational members to communicate, coordinate, process information, share resources and make joint decisions both within and across organizational boundaries [7]. Zhong et al. [8] studied the application of collaboration in knowledge management from the perspective of the supply chain and found that collaboration in supply chain knowledge management is to integrate knowledge resources and coordinate various systems within the supply chain and promote the realization of knowledge management goals. Fatfouta et al. [9] proposed a framework of an integrated and collaborative knowledge management framework that integrates the developed ontology as a knowledge model which aims to support simulation-aided design. A decentralized collaborative knowledge management was proposed to support the collaboration on RDF datasets by Natanael et al. [10]. From the perspective of collaboration technology, collaborative activities in knowledge management are mainly the process of generating knowledge achievements with a new and stable structure through the exchange and cooperation of individual behaviors with self-organization status in project management. Communication and sharing of ideas make knowledge management collaborative in nature. Collaborative knowledge management strengthens collaboration through knowledge sharing, lesson learning, problem-solving feedback, and colleagues and communities helping [11]. Collaborative knowledge management emphasizes communication and cooperation and pays more attention to the impact of collective behavior on individual behavior, as well as the knowledge results that collective behavior can produce. The collaboration will act upon the whole process of knowledge management to maximize the role of knowledge management.
Collaboration in knowledge management is mainly to study how to effectively use information technology for knowledge sharing and experience sharing, especially expert knowledge sharing management with communication as the core will be paid more and more attention by researchers [12]. In the collaborative environment of scientific and technological innovation, the use of collaboration technology can effectively solve the problems of lack of technical support and difficulties in resource sharing and process evaluation in the process of scientific research collaboration, as to promote the development of scientific research activities [13]. Reference [14] shows the evolution of cyber-physical infrastructures now offers a way of changing some early assumptions about how knowledge might be captured and displayed. Although many collaborative knowledge management methods and frameworks have been proposed in previous studies, they do not propose specific methods to support collaboration, but only stay at the conceptual level, with no specific means of implementation and evaluation.
2.2 Influence of Reciprocity Theory on Knowledge Management
According to Herbert A. Simon’s “bounded rationality” theory, behavioral economics believes that the economic behavior of economic individuals is dominated by complex motives. There are some concepts of fairness, reciprocity, and altruism in such motives, which are reciprocity preferences. Reciprocal preferences reflected in social and economic activities include (1) willingness to sacrifice their welfare to help those who are friendly to themselves; (2) Willingness to sacrifice their welfare to punish those who are not friendly to themselves [15].
Therefore, through the positive application of the reciprocal preference of the organization, we can effectively overcome the obstacles of tacit knowledge transferred between individuals in the organization and improve the efficiency of tacit knowledge transfer. Some research shows that the reciprocal cultural construction and reciprocal behavior of Research and Development (R&D) teams can significantly promote knowledge socialization and knowledge implicit [16]. In the domain of teaching, reciprocal peer tutoring is used by structured exchange of the tutor role among peers and enables each student to experience the specific benefits derived from providing and receiving academic guidance [17].
The process of knowledge sharing in knowledge management activities is essentially a reciprocal behavior, and its reciprocal motivation conforms to the “bounded rationality” hypothesis of behavioral economics for economic individuals. In the application of an online professional community could, a knowledge seeker’s intention to reciprocate is motivated by perceived social benefits. Knowledge seekers take perceived social benefits and perceived community support into account when forming an intention to reciprocate [18]. In team cooperation, an important means to promote knowledge sharing is to cultivate members’ sense of cooperation. From the perspective of reciprocity theory, the reciprocity preference of individual members always exists, and the key is how to find and use it. Therefore, in the process of knowledge sharing, the means to promote knowledge sharing can be promoted from two aspects. The first one is to build a situation of reciprocal behavior, such as establishing a good cooperation environment, technology platform, etc. The second is to evaluate the reciprocal preference of individuals, to provide support for selecting team members with reciprocal preference and building a reciprocal team.
2.3 Connotation of Reciprocal and Collaborative Knowledge Management
From the analysis of the impact of collaboration technology and reciprocity theory on knowledge management, it can be concluded that collaboration technology is conducive to the transmission and sharing of knowledge in the modern information technology environment. Collaboration technology not only improves the transmission speed of explicit knowledge but also provides a communication platform for the diffusion of tacit knowledge. Reciprocity theory provides a motive basis for the diffusion of tacit knowledge that is to promote the transfer of tacit knowledge by identifying and encouraging the reciprocal preference of tacit knowledge diffusion subjects.
Under the environment of modern information technology, Reciprocal and Collaborative Knowledge Management (RCKM) can be understood as making full use of modern information technology, taking reciprocity theory and collaboration theory as the guidance, team building as the core, cloud computing technology as the basis, serving the whole process of knowledge innovation, knowledge storage, knowledge sharing and knowledge application of various businesses of the organization.
Modern information technology is the main means of knowledge management. In the early stage of knowledge management, the knowledge base was mainly built. But with the development of modern information technology, many new technical means have emerged, especially cloud computing. Cloud computing is the latest distributed computing paradigm and it offers tremendous opportunities to solve large-scale scientific problems [19]. The reciprocity theory provides a theoretical basis for collaborative knowledge management, especially for team building. Team building is the core of collaborative knowledge management. Team building based on reciprocity theory is the institutional basis for collaborative knowledge management. Based on the hypothesis of “economic man”, learning organizations need to constantly increase incentives to keep members sharing knowledge and promote organizational progress. However, this strong incentive has also proved unsustainable in knowledge management. Therefore, under the guidance of reciprocity theory, the construction of a learning organization focuses on cultivating a reciprocal team culture, instead of strong incentives. Only when knowledge management subjects work together effectively, can the collaboration of knowledge management objects be realized [20]. When members have the characteristics of reciprocity, they will spontaneously help each other, share knowledge, and cooperate to achieve the predetermined goals. Cloud computing technology provides members with collaborative technical means to realize file resource sharing, information exchange, and traceability of the collaborative process.
The study analyzes the current problems in the research and application of knowledge management by literature research and discusses the theoretical basis for solving the problems. Based on the method of software engineering, a reciprocal and collaborative knowledge management framework based on cloud computing is constructed. This paper proposes an evaluation method of reciprocity preference and tacit knowledge based on the Bayesian method and simulates it with simulation data.
According to the theory of computer collaborative support work and reciprocity, this paper plans to design a cloud computing model for reciprocal and collaborative knowledge management. The model can be closely combined with business processes, innovate the evaluation methods of tacit knowledge and reciprocal preferences, and support the application of knowledge management. This paper introduces collaborative theory and cloud computing technology to promote the integration of knowledge management and business processes. In the current form of Economic development, knowledge has become the core element of industry-university research cooperation innovation, and knowledge management is the core content of industry-university research cooperation [21]. On the other hand, collaborative innovation has gradually become the main driving force of development. Collaborative innovation is a new innovation mode in the construction of the innovation system, which mainly solves the problems of effectiveness, efficiency, and lack of effect in collaborative innovation [22]. The research of knowledge management also began to pay more attention to the innovation and collaborative development of knowledge. As an emerging information infrastructure, cloud computing technology is receiving more and more attention. Cloud computing technology provides a strong computing platform that could support massive data storage and big data intelligent applications in different fields [23]. Cloud computing can be used in data processing, such as acquisition, storage, analytics, and visualization [24]. The services of cloud computing include software as a service (SaaS), platform as a service (PaaS), and infrastructure as a service (IaaS). SaaS provides different applications to users in the form of services according to a certain software service agreement. PaaS provides a development environmental platform to users and provides a set of application development platforms or solutions to users in the form of service. IaaS provides various network equipment, servers, databases, storage, and other facilities to users in the form of services [25,26]. At the same time, reciprocity theory is introduced to provide motive power support for collaborative behavior. The development of new-generation information technology provides a solid means of collaboration technology for the application of knowledge management. However, the application of collaboration technology not only requires team members to have corresponding technical capabilities but also the idea of collaboration. Reciprocity theory has proved in behavioral economics that individuals or organizations will be willing to bear short-term personal losses for greater common interests. Collaboration is reciprocal, so reciprocity theory can provide motive power support for collaborative behavior. The research on games shows more reciprocal players had higher bridging social capital and a higher social status [27]. Both perceived community trust and the norm of data-sharing significantly increase scientists’ awareness of academic reciprocity, which significantly affects their data-sharing intentions [28]. Direct reciprocity can promote cooperation. And indirect reciprocity can also become an important mechanism for promoting cooperation among self-interested agents via reputation and norms [29]. In the end, the Bayesian method is applied to evaluate tacit knowledge and reciprocal preference, to achieve quantitative support for collaborative behavior and reciprocal preference. In knowledge management, reciprocal behavior is not the transmission of economic benefits, but the transmission of knowledge. Therefore, it is necessary to evaluate the tacit knowledge of individuals or organizations. Then, based on collaborative behavior, reciprocal preference evaluation is realized to provide quantitative support for the selection of team members. Tacit knowledge is the knowledge existing in the human brain, is difficult to be recorded or quantified, and is often learned in face-to-face interactions. Tacit knowledge is embedded in actions, in experience, and mobilized by the knowledge carrier in a specific context. It includes cognitive and technical elements. The cognitive component refers to an individual’s mental models, i.e., his or her beliefs and preferences. The technical component consists of technical know-how which applies to a specific context [30]. The tacit knowledge diffusion depends on the decision-making of tacit knowledge owners and the expression of explicit knowledge carriers [31]. Knowledge transfer has important implications for the success of entrepreneurial firms, innovation, and organizational form [32].
4 Cloud Computing Model for RCKM
RCKM is designed to solve the collaborative application of knowledge management in the modern information technology environment. This model integrates knowledge management and business process, making them both independent and interrelated. To realize the integration of knowledge management and business project management, the concept of project group management is introduced, and project group management is used to organize and coordinate business project management and knowledge management. At the same time, cloud computing technology provides technical support for management applications.
4.1 Framework of the Cloud Computing Model for RCKM
The cloud computing model for RCKM mainly includes project group management and cloud computing technology. Project group management realizes business project management and project knowledge management, while cloud computing technology realizes technical support for project group management and knowledge management.
Project group management is a new development of modern project management theory. Project group management is to manage the projects in a coordinated way, and to obtain greater overall benefits than that of single project management by the organization of the structure and process of related projects [33]. Fernandes et al. [34] proposed to set up a project group and Project Management Office (PGPMO) dedicated to supporting university enterprise R&D cooperation and divided its functional structure into four aspects: management, finance, communication, and quality assurance. Project group management organizes business project management and knowledge management to promote the sharing of knowledge materials among multiple projects. It can coordinate knowledge managers to participate in business projects to collect business knowledge materials, evaluates business personnel, and provides knowledge support.
The cloud computing platform realizes members’ collaborative communication, massive file storage, and sharing, and knowledge case sharing, mainly including collaborative platform, private cloud disk, knowledge base, case base, etc. Project management is the management of each specific project, including organization management, team management, monitoring and warning, and achievement management. Knowledge management is embedded in the process of project management, including knowledge base support, case management, tacit knowledge evaluation, and reciprocal preference evaluation. Knowledge base support also includes knowledge warehousing, knowledge retrieval, knowledge classification, and so on. Case management is to store knowledge achievements in the knowledge base in the form of cases, to contextualize project knowledge. In this way, with knowledge base support, knowledge managers can quickly recommend relevant knowledge support according to the current project application scenario, without requiring project members to evaluate the availability of knowledge. Thus, the way of knowledge updating can be simplified. Traditionally, knowledge updating is judged according to time or annotated by knowledge managers. With the help of case management and automatic matching according to the project scenario, there is no need to worry about the problem of knowledge expiration. The framework of the cloud computing model for RCKM is shown in Fig. 1.
Figure 1: Framework of cloud computing model for RCKM
4.1.1 Project Group Management
In the cloud computing model for RCKM, the function of project group management is to manage and coordinate multiple business projects, realize knowledge and resource sharing, and promote the development of the overall business. It mainly includes business management, financial management, communication management, and quality management. The business management module controls the overall business by managing and supervising multiple business-related projects. A business process has multiple projects. Every project is established based on the needs of the business process. The project should solve the organizational and market problems that the business process needs to face. The financial management module manages the allocation of funds. Funds are the main resources allocated among projects, which can improve the effective implementation of the project better. Funds can be allocated according to the importance of the project, organizational strategy, expected income, and other indicators. Communication management refers to the interaction and cooperation between business projects. By organizing the communication between projects, administrators can find their problems and achieve further collaboration. Communication is the core of the new generation of knowledge management. Through communication, we can promote the exchange of tacit knowledge and strengthen the construction of learning organizations. However, the effect of specific tacit knowledge transfer needs to be reflected in the actual work situation and realized through tacit knowledge evaluation. Quality management is used to establish quality assessment standards and conduct KPI assessments for the project. At the same time, it supervises the evaluation of employees’ tacit knowledge and reciprocal preference in knowledge management and incorporates them into the employee assessment system. Quality assurance should ensure that the project can achieve the expected objectives, and summarize the overall business objectives after the completion of the expected objectives of each project, to provide better guidance for the development of the business.
In the cloud computing model for RCKM, project management is the management of individual projects in the business, including organization management, team management, monitoring and warning, and result management, to ensure the success of project implementation. Organization management is about how to manage a team. Referring to the learning organization theory, the project leader establishes a project vision and project objectives and allocates the resources of the project. At the same time, it classifies the documents and other material submitted by team members and organizes various collaborative activities of the project. These collaborative activities are carried out on cloud computing platforms. The project leader organizes members to work together to solve problems and achieve expected goals by organizing collaborative activities. Team management refers to the selection and role division of team members by the project leader and the construction of team culture. Project leaders can choose members according to the needs of the team and the characteristics of members, including tacit knowledge and reciprocal preferences. Then they assign the work among the members. Each member must have a clear division of labor to determine its role, to facilitate the subsequent tacit knowledge evaluation and reciprocal preference evaluation. The knowledge manager must be a member of the project team, but its particularity is that the knowledge manager plays more of the role of supervision and knowledge base support in the process of the project. The knowledge manager puts forward suggestions to team organization, timely monitors the progress of team members, finds problems, timely gives the warning, and provides appropriate knowledge support to team members. Knowledge managers can be called to be the guides and supervisors in the team. They are not only responsible for the knowledge support services of team members, but also evaluate the various behaviors of members. Monitoring and warning are the responsibilities shared by the cloud computing platform and knowledge manager. The cloud computing platform can automatically monitor the progress of the warning project, judge the progress of the project according to the set process arrangement, timely remind project leaders and project group administrators, and strengthen schedule management. The knowledge manager timely monitors and intervenes according to the behavior of members in the project. Result management is to classify and manage the results of the completed projects. The results should include the project background, project objectives, achievements, etc. Project results will be turned into important content of the case base, and the knowledge manager needs to assist the project leader to organize the project results and sort them out according to certain requirements, to facilitate the filing and use in the case base.
In the cloud computing model for RCKM, knowledge management is to manage and utilize knowledge resources to provide knowledge support, supervision, and evaluation for business projects. It mainly includes knowledge base management, case management, tacit knowledge evaluation, and reciprocal preference evaluation. Knowledge base management has always been the core of knowledge management. The knowledge base is managed by the knowledge manager. With the identification, classification, storage, and presentation of knowledge, it is convenient to support project activities. And because the knowledge manager is a project member at the same time, it is conducive to providing support to project members at any time during the implementation of the project. With the support of the knowledge base, the knowledge manager will become the thinking tank of the whole project. Therefore, knowledge managers should have high information literacy and knowledge management ability. Case management is the management of previous project processes and experience materials. It is stored in the knowledge base and exists as a part of the knowledge base. At the same time, it is different from the conventional knowledge base. The case base provides a complete knowledge application scenario, so it has more reference value for the project. The traditional knowledge base can only provide relevant knowledge for a certain problem or theme, while the case base combines knowledge with application scenarios to directly provide a replicable experience, which is conducive to process optimization and knowledge transfer. Tacit knowledge evaluation management is used to manage the tacit knowledge rating of members and evaluate the tacit knowledge of members. The tacit knowledge assessment based on the Bayesian formula can evaluate the tacit knowledge mastery probability of employees according to their achievements and behaviors, to provide the data basis for the selection and assessment of members, which is more conducive to the identification and selection of project members. Reciprocal preference evaluation management is to manage and assess the reciprocal preference level of members, to provide the basis for performance evaluation and team member selection. The knowledge manager evaluates the mutual aid behavior of members in the collaboration platform. The identification of mutual aid behavior should be operable. Mutual aid must be completed on the collaboration platform with corresponding log records. The mutual aid behavior of employee A is not only to help employee B solve the business problems in a project but also employee A’s tacit knowledge in this field should be higher than employee B’s. High tacit knowledge owners can promote the flow of tacit knowledge to low tacit knowledge owners. Otherwise, it can only be regarded as an inspiration, rather than a transfer of tacit knowledge.
4.1.4 Cloud Computing Platform
Cloud computing platform provides scalable and high-capacity cloud services, providing a basic IT environment for RCKM. Its main functions include a collaborative platform, private cloud disk, knowledge base, and case base. The collaborative platform includes a lot of information tools based on cloud computing technology, such as E-mail, instant messaging software, etc. Seven major collaborative activities require component or collaborative system support, including explicit communication, indirect communication, coordination of actions, planning, monitoring, aid, and protection [35]. Private cloud disk achieves the functions of document management, system security, and system log auditing. The major functions of the private cloud disk include file management, shared file management, and external link management. It supports the operation of setting permissions of CRUD (Create, Retrieve, Update, and Delete) for shared files. An external link is to establish folders and files to be shared with external visitors, thereby expanding the scope of the application. The knowledge and case bases are the foundation and technical realization of the overall platform. They save and display all kinds of knowledge and cases, and provide the technical basis for knowledge management applications. Both are built on cloud computing platforms to ensure the continuous expansion of storage capacity and high availability.
The process of RCKM takes project group management as the core, including the project management process and project knowledge management process. The project management process includes the processes of creating projects, selecting members, making plans, team-working, solving problems, and providing results reports. The project knowledge management process includes the process of database support, case formation, tacit knowledge evaluation, and reciprocal preference evaluation. As shown in Fig. 2.
Figure 2: Process chart of RCKM
Project group management realizes the optimization of resource allocation through the collaboration between projects. Creating a project is the first step in the project management process. According to the needs of organizational development, setting different projects will be to achieve organizational goals in the form of a project group. The second step is to select members. The main goal of selecting members is to establish a project team. The project team should be a collection of members who can work together to achieve the project goals. The composition of the team should refer to the evaluation of members’ characteristics, selection of different types of members, and then jointly formulate team goals, build a learning organization, and achieve the improvement of their ability and the achievement of project goals in the implementation of project work. Collaborative work requires a reasonable division of labor, and members need information and make plans together. At this time, it needs the support of a knowledge base. The knowledge base provides some basic working knowledge and cases for the reference of the project team. Solving problems and providing results are the goals and keys of the project team. Project members work together to complete the corresponding work content, solve the problems that the organization needs to solve, and form the corresponding results. These problem-solving processes and corresponding results are not only recorded in the employee’s files but also used as the bases for evaluation.
With process tracing, RCKM provides the knowledge base and case base needed in the process of the project in time, a better understanding of the application scenario of the project, and provides support for a case summary. The formation of cases is to refine and summarize according to the situation of the project so that it can become a reference case and be saved in the case base. Tacit knowledge evaluation is based on the results of members and the behavior of solving problems in the project, and gives the probability of tacit knowledge, to provide the basis for the next project team composition. Reciprocity preference evaluation is to calculate the reciprocity preference of members according to the mutual aid behavior of members in the process of the project, to calculate the probability of reciprocity preference, and provide one reference factor for the next team composition.
A team should be built by members with high probabilities of mastering tacit knowledge and reciprocity preference. All members are capable of completing tasks by themselves and spontaneously helping others complete project tasks. The member with a high probability of mastering tacit knowledge and a low probability of reciprocity preference can only be completed independently, but cannot help others, which is not conducive to the cultivation of new members. The members with a low probability of mastering tacit knowledge and a high probability of reciprocity preference are keen to cultivate new members. Such members are very helpful in the cultivation of new members. At the same time, they are also in the process of gradually improving tacit knowledge and need to further cultivate professional ability. The members with a low probability of mastering tacit knowledge and a low probability of reciprocity preference are new members or incompetent members, which need to be carefully identified and adjusted in time.
When the project is completed, the knowledge manager needs to catalog, classify and save the project results according to the project results data, and write the name, summary, keywords, and other information for the project cases. The abstract mainly includes four contents: application scenarios, main technologies and methods, implementation points, and results. Keywords are used to analyze the word frequency of document results, and to obtain key information for subsequent retrieval.
5 Tacit Knowledge and Reciprocal Preference Evaluation Method
5.1 Tacit Knowledge Evaluation Method
Tacit knowledge refers to action-oriented knowledge, which is procedural. Its acquisition generally does not need the help of others, and it can promote individuals to achieve their own value goals [36]. Tacit knowledge in scientific research teams is the key source of improving individual competitive advantage [37]. In high-tech enterprises, tacit knowledge is the main intellectual capital of R&D teams [38].
Tacit knowledge is unspeakable and does exist. For example, the attitude of experts in the evaluation, the doctors’ understanding of patients’ conditions, and the teachers’ evaluation of students’ learning state all affect the choice of follow-up behavior. Although tacit knowledge cannot be expressed explicitly, it will produce practical results. There are also many studies on the measurement methods of tacit knowledge transfer. The learning effect of tacit knowledge can be measured by learning rate and learning degree. The learning rate reflects learning efficiency, the learning degree describes the level of knowledge growth, and the task is the external key factor to stimulating tacit knowledge learning. However, it only expresses the classification assumption of tacit knowledge stock and increment and does not give the actual measurement method. Its research method of promoting tacit knowledge learning through tasks is very enlightening [39]. An index system for the evaluation of College Teachers’ tacit knowledge-sharing ability was built by designed questions, determined weights, and selection methods [40]. In the above research, the evaluation and measurement of tacit knowledge are mainly based on tacit knowledge-sharing models and indicators, and there is a lack of evaluation and measurement of tacit knowledge itself. This paper uses BKT knowledge tracing theory [41,42] for reference and uses the Bayesian formula to calculate the probability of tacit knowledge mastery.
Because tacit knowledge itself is not visible, and there is no certain standard, it can only be measured from the process or result of the transfer, rather than directly measured. The purpose of knowledge transfer is knowledge innovation. The innovative achievements are obvious and explicit. Therefore, it is feasible to evaluate tacit knowledge with knowledge innovation achievements and behaviors.
In RCKM, the tacit knowledge evaluation of members is mainly calculated by the knowledge manager through the Bayesian formula, such as Eq. (1), according to the results and problem-solving of project members.
Through calculation, the tacit knowledge mastery probability of members in this field is obtained, to provide references for future project team organization. According to the Bayesian formula, tacit knowledge mastery is defined as event B, and the behavior of using tacit knowledge to solve problems is defined as event A. Calculate the probability of B by the count of occurrences of event A, to obtain a relatively accurate probability of employees’ tacit knowledge mastery. Taking a software project as an example, the event that the software project passes the acceptance is A, and the tacit knowledge in the project field is B, as shown in Table 1.
This probability assumption is in line with the actual situation. Software project managers with more tacit knowledge of the specific field have a higher probability of completing a project. Managers with little tacit knowledge of the specific field have a very low probability of completing a project. So, a very low probability can be set for the initial tacit knowledge state, indicating that it is very difficult. Then calculate the tacit knowledge of the managers according to the count of completed software projects. The calculation results between the count of completed projects and the mastery of tacit knowledge in the field are shown in Table 2.
It can be seen that if it is difficult to complete, the probability of mastering the tacit knowledge in this field can be confirmed to be 1 with more count than three completed projects. Similarly, the connotation of tacit knowledge in the representation field is the same when the completed project is transformed into the solution to core problems.
From the above calculation, it can be found that if the correlation between event A and event B is regarded as the process of transforming tacit knowledge into actual results, and this transformation is defined as the transformation probability, there are two states of tacit knowledge mastery in tacit knowledge evaluation, namely, mastery and non-mastery, then Eq. (1) can be simplified to a more targeted Eq. (2).
n >= 1,
This Eq. (2) is derived based on the Bayesian formula, which is used to calculate the mastery state of tacit knowledge. It can be used to evaluate the tacit knowledge of organization members, effectively organize teams, and carry out performance evaluations. The operation diagram of this method is shown in Fig. 3. The evaluation of tacit knowledge is based on evidence, which comes from various achievements in the cloud platform, such as reports, project experiences, articles, books, etc.
Figure 3: Operation diagram of tacit knowledge evaluation
5.2 Reciprocal Preference Evaluation Method
When individuals think that knowledge belongs to individuals rather than teams, they will be exclusive. Individuals think that sharing knowledge will weaken their competitive advantage and inhibit their willingness to share knowledge [43]. From the view of technology, collaborative filtering has been a widely used technique in recommender systems. It generates recommendations by leveraging the user-item interactions derived from historical data [44]. At the same time, the collaborative activity requires a structured and open environment where individuals can actively exchange and elaborate their ideas to achieve a high-quality problem-solving solution. The use of a concept map has been extensively implemented to facilitate idea generation and maintain shared focus during discussion [45]. Therefore, teams need to build a reciprocal culture and improve individual reciprocal preferences. The identification of members’ reciprocal preferences is mainly to judge members’ reciprocal preferences through the mutually supportive behaviors among members in the cloud computing platform. Analogically, reciprocal preference can also establish quantitative indicators. Similar to tacit knowledge evaluation, reciprocity preference probability can be calculated by the Bayesian formula. Therefore, based on the Bayesian formula, the same derivation method as Eq. (2) is used to derive Eq. (3) to calculate the probability of reciprocity preference.
n >= 1,
Specifically, assuming that members have reciprocal preferences, the probability is
For example, in an enterprise, when evaluating employees’ reciprocal preferences, according to the assumptions in the above table,
It can be seen from the above table that the more mutual aid, the higher the probability of reciprocity preference. When the count of mutual aid reaches 30, the reciprocity preference probability can reach 1. When a member’s probability equals 1, it can recognize that he has a complete reciprocity reference. Therefore, selecting team members with reciprocal preferences by reciprocity evaluation will help to improve the performance of tacit knowledge sharing. The operation diagram of this method is shown in Fig. 4. Reciprocity preference evaluation is based on evidence that comes from various records in the cloud platform, such as mutual aid logs, number of file shares, discussion records, guidance records, etc.
Figure 4: Operation diagram of reciprocity preference evaluation
The data used in the reciprocity preference evaluation mainly comes from various records and logs of the cloud platform. Therefore, organizational members should be encouraged to actively use various collaboration tools of the cloud platform to collect behavioral data of organizational members naturally. At the same time, it is needed to form online records of all kinds of offline seminars, exchanges, and discussions, which are not only conducive to analysis and backtracking but also convenient for evaluation. In this process, knowledge managers should play a more active role in changing business work from offline to online.
RCKM takes collaboration as the core and carries out knowledge management with the basis of cloud computing technology, breaking through the traditional problem of separating knowledge management from business processes. Through project team management, the collaborative behavior of the organization members can be strengthened, which is conducive to gradually forming an advantageous project team, highlighting the organizational characteristics, and establishing a dominant position in a certain field. The collaborative characteristics of RCKM are reflected in the synchronization between project and knowledge management and the collaboration in the process of the project itself, which organically integrates project management and knowledge management. On the other hand, the collaboration between members is not only reflected in the work, but also the assessment. Assessment makes members more inclined to help colleagues so that while maximizing the overall interests, individuals can also obtain personal benefits in the collaboration. Mutual benefits between individuals and between individuals and organizations are conducive to the establishment of a close cooperative learning organization.
For the application and implementation of RCKM in various organizations, this paper puts forward suggestions from four aspects, e.g., strengthening collaborative management, strengthening the capacity-building of knowledge managers, strengthening the construction of reciprocal teams, and strengthening the integration of knowledge management and business processes.
Strengthen collaborative capacity by adopting project group management, and promote the development of organizational members. At present, few enterprises and organizations adopt project group management, and most of them complete the corresponding work with project management. For organizational members, the completion of a project has no other benefits except the improvement of experience and the completion of performance appraisal. But project group management is conducive to promoting the cognition of the whole project group. If the project group is advantageous, it has more room for members’ growth and development. If the project group is weak, members need to consider how to make it stronger; if it can’t become stronger, they should change their thoughts as soon as possible, improve their ability, and move closer to the advantageous project group.
Strengthen the capacity-building of knowledge managers to give better play to the role of knowledge management, and improve the level of knowledge sharing within the organization. In RCKM, knowledge managers play an important role. They perform many functions in the team, such as think tanks, experts, supervisors, and so on. They should give full play to their role and set up a separate role in the project organization to complete the task of knowledge management. But the knowledge managers do not undertake specific tasks during the completion of the projects. So there is a risk of marginalization for them. Therefore, it is necessary to solidify its responsibilities, and then the knowledge manager can guide the work of members, rather than sit on the sidelines and just be a spectator. Moreover, knowledge managers have the authority of human resource management, can evaluate employees’ tacit knowledge and reciprocal preferences, and therefore have independent team functions. And because the main role of knowledge managers is to provide knowledge management, they can participate in multiple teams at the same time.
Strengthen the construction of reciprocal teams to let team members share knowledge, promote knowledge transfer, and improve team efficiency in a favorable atmosphere of mutual aid. In the construction of reciprocal teams, it should strengthen cultural construction, cultivate a good working atmosphere and sharing atmosphere, and make members help each other to improve efficiency. There are spontaneous communities of practice in technology-based small and medium-sized enterprises. Such enterprises should actively promote the development of practice communities and establish a cultural atmosphere of trust and reciprocity, to improve work efficiency and obtain more social wealth [46].
Strengthen the integration of knowledge management and business processes to improve organizational performance. Integrating with management information systems and deploying an incentive mechanism to trigger stakeholders’ participation in using/reusing knowledge resources are useful to promote organizational performance [47]. Based on the project mechanism, the workflow is decomposed and organically integrated with the knowledge management process, so that the project process can be tracked. The work of project management and knowledge management is an organic whole. Traditionally knowledge management is taken as an auxiliary work and now as a part of a necessary work, to improve overall business efficiency. Infiltrate knowledge management into the project process, and further refine it with the support of cloud computing technology, to provide better support for the development and evaluation of members.
The limitation of this paper lies in how the reciprocal preference and tacit knowledge evaluation value promote each other with the existing personnel evaluation system in practical application, as well as the specific technical means for obtaining the evaluation data, and the use of the evaluation data.
The future work is to make more applications in enterprises and organizations, and use big data analysis to further explore the relationship between reciprocal preferences, tacit knowledge, and individual performance levels. On the other hand, it is necessary to research to build a corresponding management system and rules so that the knowledge management professionals in the human resources department can manage and collect relevant evaluation data.
Funding Statement: This paper is supported by the research project of the Jiangsu water conservancy science and technology project (Contract Number: 2021067).
Conflicts of Interest: The authors declare that they have no conflicts of interest to report regarding the present study.
References
1. Z. W. Lin, T. J. Zhang and Y. J. Pu, “The study on the tacit knowledge transition of person-to-person based on reciprocity motivation,” Science Research Management, vol. 29, no. 4, pp. 28–33, 2008. [Google Scholar]
2. G. F. Yi, “A study on influence of knowledge management strategy on performance of academic entrepreneurship team in universities——the construction of digital knowledge management platform,” Forum on Science and Technology in China, vol. 34, no. 12, pp. 173–181, 2018. [Google Scholar]
3. V. Costa and S. Monteiro, “Key knowledge management processes for innovation: A systematic literature review,” VINE Journal of Information and Knowledge Management Systems, vol. 46, no. 3, pp. 386–410, 2016. [Google Scholar]
4. M. Zanker and V. Bureš, “Knowledge management as a domain, system dynamics as a methodology,” Systems, vol. 10, no. 3, pp. 82, 2022. [Google Scholar]
5. X. Zhang, Y. L. Li, Y. H. Cheng and H. S. Zhu, “Framework, theory, and practice of digitalized knowledge management: A survey,” Frontiers of Data & Computing, vol. 3, no. 2, pp. 23–38, 2021. [Google Scholar]
6. J. F. Su, Y. Yang and N. Zhang, “Study on the tacit knowledge diffusion in knowledge collaboration team based on heterogeneous cellular automata,” Chinese Journal of Management, vol. 14, no. 2, pp. 245–253, 2017. [Google Scholar]
7. H. Bala, A. P. Massey and M. M. Montoya, “The effects of process orientations on collaboration technology use and outcomes in product development,” Journal of Management Information Systems, vol. 34, no. 2, pp. 520–559, 2017. [Google Scholar]
8. Y. H. Zhong and X. P. Chen, “Evolutionary game analysis of low carbon supply chain based on collaborative knowledge management perspective,” Science and Technology Management Research, vol. 34, no. 3, pp. 214–217, 2014. [Google Scholar]
9. N. Fatfouta and J. Stal-Le Cardinal, “Towards a framework for integrated and collaborative knowledge management for engineering design–a case study,” in Proc. Design Conf., Virtual, Online, vol. 1, pp. 559–568, 2020. [Google Scholar]
10. A. Natanael, N. Patrick, R. Norman, M. Michael and M. Edgard, “Decentralized collaborative knowledge management using git,” Journal of Web Semantics, vol. 54, pp. 29–47, 2019. [Google Scholar]
11. B. Komal, R. Mariam, B. Afnan and K. Faria, “Designing and evaluating a collaborative knowledge management framework for leaf disease detection,” Computer Systems Science and Engineering, vol. 42, no. 2, pp. 751–777, 2021. [Google Scholar]
12. M. S. Ackerman, J. Dachtera, V. Pipek and V. Wulf, “Sharing knowledge and expertise: The CSCW view of knowledge management,” Computer Supported Cooperative Work (CSCW), vol. 22, no. 4, pp. 531–573, 2013. [Google Scholar]
13. J. Q. Li, Y. J. Bian and F. Guo, “Innovation of cooperative mechanism of scientific research based on the computer-supported cooperative work,” Science and Technology Management Research, vol. 39, no. 23, pp. 182–187, 2019. [Google Scholar]
14. A. F. P. de Carvalho, S. Hoffmann, D. Abele, M. Schweitzer, P. Tolmie et al., “Of embodied action and sensors: Knowledge and expertise sharing in industrial set-up,” Computer Supported Cooperative Work (CSCW), vol. 27, no. 3–6, pp. 875–916, 2018. [Google Scholar]
15. M. Rabin, “Incorporating fairness into game theory and economics,” The American Economic Review, vol. 23, no. 5, pp. 1281–1302, 1993. [Google Scholar]
16. T. J. Zhang and Y. J. Pu, “Research on the correlation between reciprocity preference, tacit knowledge transformation and technological innovation ability,” Management Review, vol. 22, no. 10, pp. 100–106, 2010. [Google Scholar]
17. L. D. Backer, H. V. Keer, B. Moerkerke and M. Valcke, “Examining evolutions in the adoption of metacognitive regulation in reciprocal peer tutoring groups,” Metacognition Learning, vol. 11, no. 2, pp. 187–213, 2016. [Google Scholar]
18. C. A. Tsai and T. C. Kang, “Reciprocal intention in knowledge seeking: Examining social exchange theory in an online professional community,” International Journal of Information Management, vol. 48, pp. 161–174, 2019. [Google Scholar]
19. M. A. Rodriguez and R. Buyya, “Deadline based resource provisioning and scheduling algorithm for scientific workflows on clouds,” IEEE Transactions on Cloud Computing, vol. 2, no. 2, pp. 222–235, 2014. [Google Scholar]
20. H. M. Shen and Q. Ke, “Integration of knowledge management technology and collaborative technology,” Science & Technology Progress and Policy, vol. 30, no. 1, pp. 136–139, 2013. [Google Scholar]
21. Z. Su, “Conflict analysis and countermeasure of industry-university-research cooperation innovation from the perspective of knowledge management,” Science & Technology Progress and Policy, vol. 35, no. 24, pp. 70–76, 2018. [Google Scholar]
22. X. M. An, W. L. Bai and W. R. Zhong, “Knowledge management approaches to support of community capacity design,” Science & Technology Progress and Policy, vol. 32, no. 6, pp. 136–141, 2015. [Google Scholar]
23. Y. Ding, Y. Shao, J. Zhang, X. Nie, P. Yang et al., “A cloud computing platform technology based on space-based intelligent information network,” in Proc. ICRAIC, Wuhan, China, pp. 1–5, 2021. [Google Scholar]
24. F. Rui, G. Feng, Z. Rong, J. Hu and Q. Lu, “Big data and cloud computing platform for energy internet,” in China Int. Electrical and Energy Conf. (CIEEC), Beijing, China, pp. 681–686, 2017. [Google Scholar]
25. Y. Hu, “Library information resource sharing cloud service based on cloud computing mode,” Journal of Intelligent and Fuzzy Systems, vol. 37, no. 5, pp. 5867–5875, 2019. [Google Scholar]
26. Z. Lv, “Design of cross-source education information classification model based on cloud computing technology,” Advances in Multimedia, vol. 2022, pp. 1–12, 2022. [Google Scholar]
27. S. S. Y. Kim, K. M. Huang-Isherwood, W. Zheng and D. Williams, “The art of being together: How group play can increase reciprocity, social capital, and social status in a multiplayer online game,” Computers in Human Behavior, vol. 133, no. 3, pp. 1–11, 2022. [Google Scholar]
28. Y. Kim, “Reputation, trust, and norms as mechanisms forming academic reciprocity in data sharing: An empirical test of theory of collective action,” Aslib Journal of Information Management, vol. 74, no. 6, pp. 1174–1195, 2022. [Google Scholar]
29. K. Marcus and T. A. Han, “Pleasing enhances indirect reciprocity-based cooperation under private assessment,” Artificial Life, vol. 27, no. 3, pp. 246–276, 2021. [Google Scholar]
30. P. E. Arduin, M. Grundstein, B. Mayag, E. Negre, C. Rosenthal-Sabroux et al., “The importance of tacit knowledge when teaching suddenly online,” in Proc. ICIKS, Virtual, Online, vol. 425, pp. 29–42, 2021. [Google Scholar]
31. L. Song and Y. H. Ma, “Evaluating tacit knowledge diffusion with algebra matrix algorithm based social networks,” Applied Mathematics and Computation, vol. 428, pp. 1–20, 2022. [Google Scholar]
32. L. Argote and E. Fahrenkopf, “Knowledge transfer in organizations: The roles of members, tasks, tools, and networks,” Organizational Behavior and Human Decision Processes, vol. 136, no. 3, pp. 146–159, 2016. [Google Scholar]
33. D. C. Ferns, “Development in programme management,” International Journal of Project Management, vol. 9, no. 3, pp. 148–156, 1991. [Google Scholar]
34. G. Fernandes, E. B. Pinto, M. T. Araújo and R. J. Machado, “The roles of a programme and project management office to support collaborative university-industry R&D,” Total Quality Management & Business Excellence, vol. 31, no. 5–6, pp. 538–608, 2020. [Google Scholar]
35. C. Gutwin and S. Greenberg, “The mechanics of collaboration: Developing low cost usability evaluation methods for shared workspaces,” in IEEE Int. Workshops on Enabling Technologies: Infrastructure for Collaborative Enterprises, Gaithersburg, MD, USA, IEEE Computer Society, pp. 98–103, 2000. [Google Scholar]
36. R. J. Stemberg, G. B. Forsythe, J. Hedlund, J. A. Horvath, R. K. Wagner et al., Practical intelligence in everyday life. New York, USA: Cambridge University Press, 2000. [Google Scholar]
37. M. Zhang, G. Q. Tang and Y. Zhang, “Influencing factors of tacit knowledge sharing desire in university research team,” Information Studies: Theory & Application, vol. 40, no. 6, pp. 82–86, 2017. [Google Scholar]
38. Y. Zhang, T. J. Zhang and B. Chen, “The correlation study among skinner reinforcement incentives, internal knowledge transformation and organizational creativity-based on the data certification of research and development team of high-tech enterprises in Yangtze River Delta,” Science and Technology Management Research, vol. 38, no. 1, pp. 141–148, 2018. [Google Scholar]
39. N. Xia and B. Hu, “A research on task-driven learning of tacit knowledge,” Science Research Management, vol. 37, no. 5, pp. 141–149, 2016. [Google Scholar]
40. D. Z. Sun, Y. P. Li and D. K. Yu, “Ability evaluation model of university teachers’ tacit knowledge sharing,” Information Science, vol. 33, no. 4, pp. 46–52, 2015. [Google Scholar]
41. A. T. Corbett and J. R. Anderson, “Knowledge tracing: Modeling the acquisition of procedural knowledge,” User Modeling and User-Adapted Interaction, vol. 4, no. 4, pp. 253–278, 1994. [Google Scholar]
42. J. Q. Li, Y. J. Bian and Z. Fang, “Research on tracing evaluation of web-based teaching based on BKT model,” Modern Distance Education Research, vol. 155, no. 5, pp. 106–114, 2018. [Google Scholar]
43. R. J. Liu, Q. Zhang and W. Min, “Research on the relationship between psychological ownership of knowledge and tacit knowledge sharing,” Science & Technology Progress and Policy, vol. 30, no. 9, pp. 134–138, 2013. [Google Scholar]
44. R. Yu, Q. Liu, Y. Ye, M. Cheng, E. Chen et al., “Collaborative list-and-pairwise filtering from implicit feedback,” IEEE Transactions on Knowledge and Data Engineering, vol. 34, no. 6, pp. 2667–2680, 2022. [Google Scholar]
45. L. Sadita, T. Hirashima, Y. Hayashi, P. G. F. Furtado, K. Junus et al., “The effect of differences in group composition on knowledge transfer, group achievement, and learners’ affective responses during reciprocal concept mapping with the Kit-Build Approach,” Research and Practice in Technology Enhanced Learning, vol. 15, no. 13, pp. 1, 2020. [Google Scholar]
46. S. Pattinson and D. Preece, “Communities of practice, knowledge acquisition and innovation: A case study of science-based SMEs,” Journal of Knowledge Management, vol. 18, no. 1, pp. 107–120, 2014. [Google Scholar]
47. X. Y. Li, C. H. Chen, P. Zheng, Z. H. Jiang and L. K. Wang, “A context-aware diversity-oriented knowledge recommendation approach for smart engineering solution design,” Knowledge-Based Systems, vol. 215, pp. 1–16, 2021. [Google Scholar]
Cite This Article
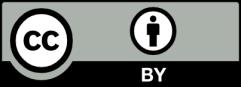
This work is licensed under a Creative Commons Attribution 4.0 International License , which permits unrestricted use, distribution, and reproduction in any medium, provided the original work is properly cited.