Open Access
ARTICLE
Deep Learning Method to Detect the Road Cracks and Potholes for Smart Cities
1 College of Civil Engineering, Hunan University, Changsha, 410082, China
2 Department of CS, University of Okara, Okara, 56310, Pakistan
3 Department of CS&SE, Islamic International University, Islamabad, 44000, Pakistan
4 Department of IT Services, University of Okara, Okara, 56310, Pakistan
5 Department of CS, Government College University, Faisalabad, 38000, Pakistan
6 Department of Automation Science, Beihang University, Beijing, 100191, China
* Corresponding Author: Javed Rashid. Email:
Computers, Materials & Continua 2023, 75(1), 1863-1881. https://doi.org/10.32604/cmc.2023.035287
Received 15 August 2022; Accepted 17 November 2022; Issue published 06 February 2023
Abstract
The increasing global population at a rapid pace makes road traffic dense; managing such massive traffic is challenging. In developing countries like Pakistan, road traffic accidents (RTA) have the highest mortality percentage among other Asian countries. The main reasons for RTAs are road cracks and potholes. Understanding the need for an automated system for the detection of cracks and potholes, this study proposes a decision support system (DSS) for an autonomous road information system for smart city development with the use of deep learning. The proposed DSS works in layers where initially the image of roads is captured and coordinates attached to the image with the help of global positioning system (GPS), communicated to the decision layer to find about the cracks and potholes in the roads, and eventually, that information is passed to the road management information system, which gives information to drivers and the maintenance department. For the decision layer, we projected a CNN-based model for pothole crack detection (PCD). Aimed at training, a K-fold cross-validation strategy was used where the value of K was set to 10. The training of PCD was completed with a self-collected dataset consisting of 6000 images from Pakistani roads. The proposed PCD achieved 98% of precision, 97% recall, and accuracy while testing on unseen images. The results produced by our model are higher than the existing model in terms of performance and computational cost, which proves its significance.Keywords
Cite This Article
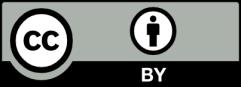
This work is licensed under a Creative Commons Attribution 4.0 International License , which permits unrestricted use, distribution, and reproduction in any medium, provided the original work is properly cited.