Open Access
ARTICLE
Energy Prediction in IoT Systems Using Machine Learning Models
Department of ECE, SRM Institute of Science and Technology, Vadapalani Campus, No1 Jawaharlal Nehru Road, Vadapalani, 600026, TN, India
* Corresponding Author: S. Balaji. Email:
Computers, Materials & Continua 2023, 75(1), 443-459. https://doi.org/10.32604/cmc.2023.035275
Received 15 August 2022; Accepted 24 October 2022; Issue published 06 February 2023
Abstract
The Internet of Things (IoT) technology has been developed for directing and maintaining the atmosphere in smart buildings in real time. In order to optimise the power generation sector and schedule routine maintenance, it is crucial to predict future energy demand. Electricity demand forecasting is difficult because of the complexity of the available demand patterns. Establishing a perfect prediction of energy consumption at the building’s level is vital and significant to efficiently managing the consumed energy by utilising a strong predictive model. Low forecast accuracy is just one of the reasons why energy consumption and prediction models have failed to advance. Therefore, the purpose of this study is to create an IoT-based energy prediction (IoT-EP) model that can reliably estimate the energy consumption of smart buildings. A real-world test case on power predictions is conducted on a local electricity grid to test the practicality of the approach. The proposed (IoT-EP) model selects the significant features as input neurons, the predictable data is selected as output nodes, and a multi-layer perceptron is constructed along with the features of the Convolution Neural Network (CNN) algorithm. The analysis of the proposed IoT-EP model has higher accuracy of 90%, correlation of 89%, and variance of 16% in less training time of 29.2 s, and with a higher prediction speed of 396 (observation/sec). When compared to existing models, the results showed that the proposed (IoT-EP) model outperforms with a satisfactory level of accuracy in predicting energy consumption in smart buildings.Keywords
Cite This Article
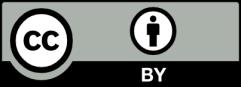
This work is licensed under a Creative Commons Attribution 4.0 International License , which permits unrestricted use, distribution, and reproduction in any medium, provided the original work is properly cited.