Open Access
ARTICLE
Expert Recommendation in Community Question Answering via Heterogeneous Content Network Embedding
1 School of Computer Science and Technology, Huazhong University of Science and Technology, Wuhan 430074, China
2 College of Computer Science and Technology, Hubei University of Technology, Wuhan 430068, China
* Corresponding Author: Hong Li. Email:
Computers, Materials & Continua 2023, 75(1), 1687-1709. https://doi.org/10.32604/cmc.2023.035239
Received 13 August 2022; Accepted 08 December 2022; Issue published 06 February 2023
Abstract
Expert Recommendation (ER) aims to identify domain experts with high expertise and willingness to provide answers to questions in Community Question Answering (CQA) web services. How to model questions and users in the heterogeneous content network is critical to this task. Most traditional methods focus on modeling questions and users based on the textual content left in the community while ignoring the structural properties of heterogeneous CQA networks and always suffering from textual data sparsity issues. Recent approaches take advantage of structural proximities between nodes and attempt to fuse the textual content of nodes for modeling. However, they often fail to distinguish the nodes’ personalized preferences and only consider the textual content of a part of the nodes in network embedding learning, while ignoring the semantic relevance of nodes. In this paper, we propose a novel framework that jointly considers the structural proximity relations and textual semantic relevance to model users and questions more comprehensively. Specifically, we learn topology-based embeddings through a hierarchical attentive network learning strategy, in which the proximity information and the personalized preference of nodes are encoded and preserved. Meanwhile, we utilize the node’s textual content and the text correlation between adjacent nodes to build the content-based embedding through a meta-context-aware skip-gram model. In addition, the user’s relative answer quality is incorporated to promote the ranking performance. Experimental results show that our proposed framework consistently and significantly outperforms the state-of-the-art baselines on three real-world datasets by taking the deep semantic understanding and structural feature learning together. The performance of the proposed work is analyzed in terms of MRR, P@K, and MAP and is proven to be more advanced than the existing methodologies.Keywords
Cite This Article
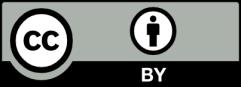