Open Access
ARTICLE
Continuous Mobile User Authentication Using a Hybrid CNN-Bi-LSTM Approach
1 Department of Information Technology, University of Tabuk, Tabuk, 71491, Saudi Arabia
2 Sensor Networks and Cellular Systems Research Center, University of Tabuk, Tabuk, 71491, Saudi Arabia
* Corresponding Author: Bandar Alotaibi. Email:
Computers, Materials & Continua 2023, 75(1), 651-667. https://doi.org/10.32604/cmc.2023.035173
Received 10 August 2022; Accepted 19 November 2022; Issue published 06 February 2023
Abstract
Internet of Things (IoT) devices incorporate a large amount of data in several fields, including those of medicine, business, and engineering. User authentication is paramount in the IoT era to assure connected devices’ security. However, traditional authentication methods and conventional biometrics-based authentication approaches such as face recognition, fingerprints, and password are vulnerable to various attacks, including smudge attacks, heat attacks, and shoulder surfing attacks. Behavioral biometrics is introduced by the powerful sensing capabilities of IoT devices such as smart wearables and smartphones, enabling continuous authentication. Artificial Intelligence (AI)-based approaches introduce a bright future in refining large amounts of homogeneous biometric data to provide innovative user authentication solutions. This paper presents a new continuous passive authentication approach capable of learning the signatures of IoT users utilizing smartphone sensors such as a gyroscope, magnetometer, and accelerometer to recognize users by their physical activities. This approach integrates the convolutional neural network (CNN) and recurrent neural network (RNN) models to learn signatures of human activities from different users. A series of experiments are conducted using the MotionSense dataset to validate the effectiveness of the proposed method. Our technique offers a competitive verification accuracy equal to 98.4%. We compared the proposed method with several conventional machine learning and CNN models and found that our proposed model achieves higher identification accuracy than the recently developed verification systems. The high accuracy achieved by the proposed method proves its effectiveness in recognizing IoT users passively through their physical activity patterns.Keywords
Cite This Article
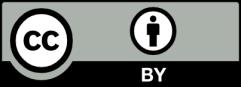