Open Access
ARTICLE
Gaussian Blur Masked ResNet2.0 Architecture for Diabetic Retinopathy Detection
1 School of Electronics Engineering, Vellore Institute of Technology, Chennai, 600127, India
2 School of Computer Science and Engineering, Vellore Institute of Technology, Chennai, 600127, India
3 Centre for Cyber-Physical Systems, Vellore Institute of Technology, Chennai, 600127, India
* Corresponding Author: Manas Ranjan Prusty. Email:
Computers, Materials & Continua 2023, 75(1), 927-942. https://doi.org/10.32604/cmc.2023.035143
Received 09 August 2022; Accepted 17 November 2022; Issue published 06 February 2023
Abstract
Diabetic Retinopathy (DR) is a serious hazard that can result in irreversible blindness if not addressed in a timely manner. Hence, numerous techniques have been proposed for the accurate and timely detection of this disease. Out of these, Deep Learning (DL) and Computer Vision (CV) methods for multiclass categorization of color fundus images diagnosed with Diabetic Retinopathy have sparked considerable attention. In this paper, we attempt to develop an extended ResNet152V2 architecture-based Deep Learning model, named ResNet2.0 to aid the timely detection of DR. The APTOS-2019 dataset was used to train the model. This consists of 3662 fundus images belonging to five different stages of DR: no DR (Class 0), mild DR (Class 1), moderate DR (Class 2), severe DR (Class 3), and proliferative DR (Class 4). The model was gauged based on ability to detect stage-wise DR. The images were pre-processed using negative and positive weighted Gaussian-based masks as feature engineering to further enhance the quality of the fundus images by removing the noise and normalizing the images. Up-sampling and data augmentation methods were used to address the skewness of the original dataset. The proposed model achieved an overall accuracy of 91% and an area under the receiver-operating characteristic curve (AUC) score of 95.1%, outperforming existing Deep Learning models by around 10%. Furthermore, the class-wise F1 score for No DR was 92%, Mild DR was 82%, Moderate DR was 66%, Severe was DR 89% and Proliferative DR was 80%.Keywords
Cite This Article
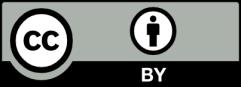