Open Access
ARTICLE
Enhanced E-commerce Fraud Prediction Based on a Convolutional Neural Network Model
1 Chenzhou Vocational Technical College, Chenzhou, 423000, China
2 Hunan University of Finance and Economics, Changsha, 410205, China
3 University Malaysia Sabah, Kota Kinabalu, 88999, Malaysia
* Corresponding Author: Ling Liu. Email:
Computers, Materials & Continua 2023, 75(1), 1107-1117. https://doi.org/10.32604/cmc.2023.034917
Received 01 August 2022; Accepted 08 December 2022; Issue published 06 February 2023
Abstract
The rapidly escalating sophistication of e-commerce fraud in recent years has led to an increasing reliance on fraud detection methods based on machine learning. However, fraud detection methods based on conventional machine learning approaches suffer from several problems, including an excessively high number of network parameters, which decreases the efficiency and increases the difficulty of training the network, while simultaneously leading to network overfitting. In addition, the sparsity of positive fraud incidents relative to the overwhelming proportion of negative incidents leads to detection failures in trained networks. The present work addresses these issues by proposing a convolutional neural network (CNN) framework for detecting e-commerce fraud, where network training is conducted using historical market transaction data. The number of network parameters reduces via the local perception field and weight sharing inherent in the CNN framework. In addition, this deep learning framework enables the use of an algorithmic-level approach to address dataset imbalance by focusing the CNN model on minority data classes. The proposed CNN model is trained and tested using a large public e-commerce service dataset from 2018, and the test results demonstrate that the model provides higher fraud prediction accuracy than existing state-of-the-art methods.Keywords
Cite This Article
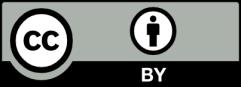